Summary: Modest UX salary drop in 2024 | Reasoning model scaling law confirmed | AI task scope doubles every 7 Months | Customer journey management | 3 new AI image generators: Reve, OpenAI native mode, Ideogram 3
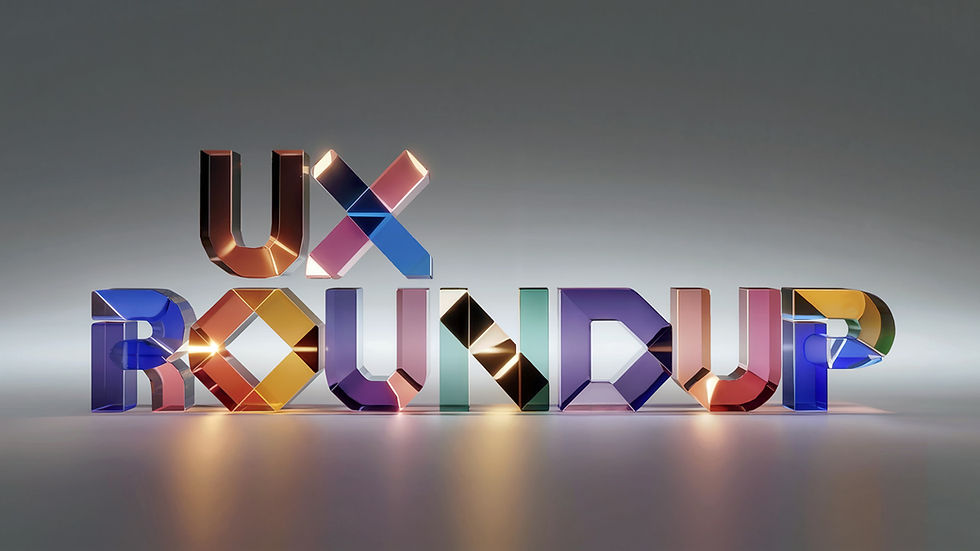
UX Roundup for March 31, 2025. (Midjourney)
Modest UX Salary Drop in 2024
After UX salaries plunged by a whopping 12% in 2023, it seems that the worst is over. The Wall Street Journal reports that the pay for product designers only decreased by between 1% and 2% during the second half of 2024. They do not report how much salaries dropped in the first half of 2024, but even if the first half of the year was a little worse than the second half, the total salary adjustment in 2024 is likely to have been around 4%.
Only a third as big a salary drop in 2024 as in 2023. I believe I have been proven right in my article, “UX Angst of 2023-24,” where I stated that the challenging times during those years were an inevitable correction following the excesses of the 2020-22 bubble years. They were not predictors of eternal doom for UX. It appears that UX hiring is picking up, even if it has not yet returned to the same levels.
Now, we’re almost certainly back to normal, with steady UX salaries. Consider that UX salaries have remained virtually unchanged for 27 years, after adjusting for inflation. The two exceptions to the flat line were the dot-com bubble and the recent bubble. People who have recently entered the UX profession (which is the majority of UX staff) may think of 2022 as normal, rather than a bubble, but in retrospect, it was a bubble relative to the broader picture of UX salaries since 1998.
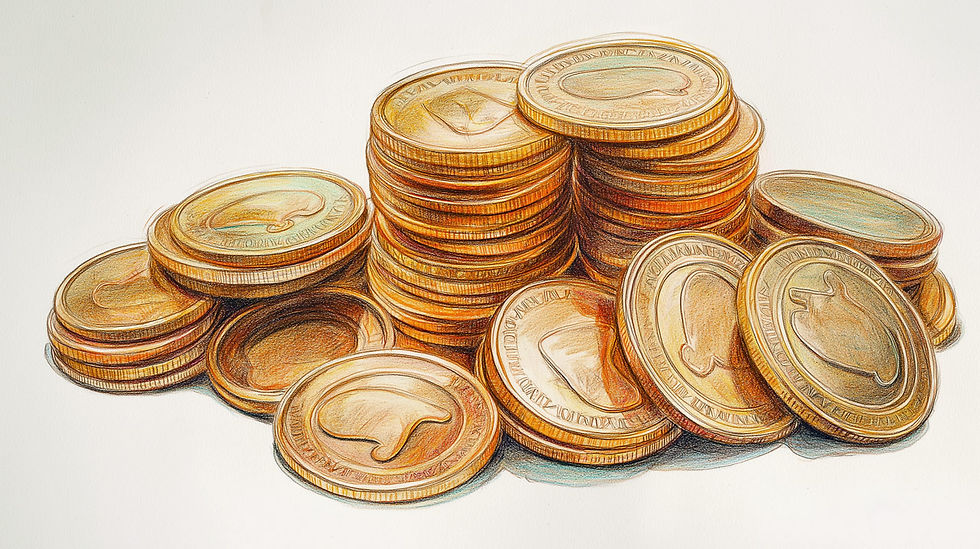
You still make good gold as a UX professional in 2025, even if 16% less than in 2022. But since current inflation-adjusted compensation is about the same as it has been for most years since 1998, I would expect good salaries to continue. (Midjourney)
Reasoning Model Scaling Law Confirmed
Epoch AI has tested GPT o3-mini on a benchmark of difficult math problems. They found that running this reasoning model multiple times increased the chance that it could solve the problem correctly at least once. The reasoning model’s performance increased by 2.6 percentage points per doubling of the number of attempts, increasing from 8.1% correct in a single attempt (remember these are difficult math problems I could not solve) to 18.3% correct in 16 attempts.
This particular outcome is only useful in cases where it can be determined whether an answer is right or wrong. This is true for math problems and also for other specialized domains such as playing chess. If you don’t know which answer is right, it does you no good to have 16 answers, because you would not be able to tell which is the right answer.
However, the study is useful because it confirms AI scaling for test-time compute and demonstrates that this scaling is logarithmic, not linear. That is, to improve performance by a certain amount, you have to double the compute investment and not just increase it by a fixed amount. So, for example, to improve by 3 steps, you must spend 8x as much compute and not just 3x.
Bottom line: thinking harder does work for AI. (Of course, we also want it to think smarter. There are several AI scaling laws, after all. And we also want AI to work for cheap, as shown by the success of DeepSeek R1. With human employees, you might feel bad for underpaying them, but for AI, let’s squeeze that sucker and pay it as little as possible.)
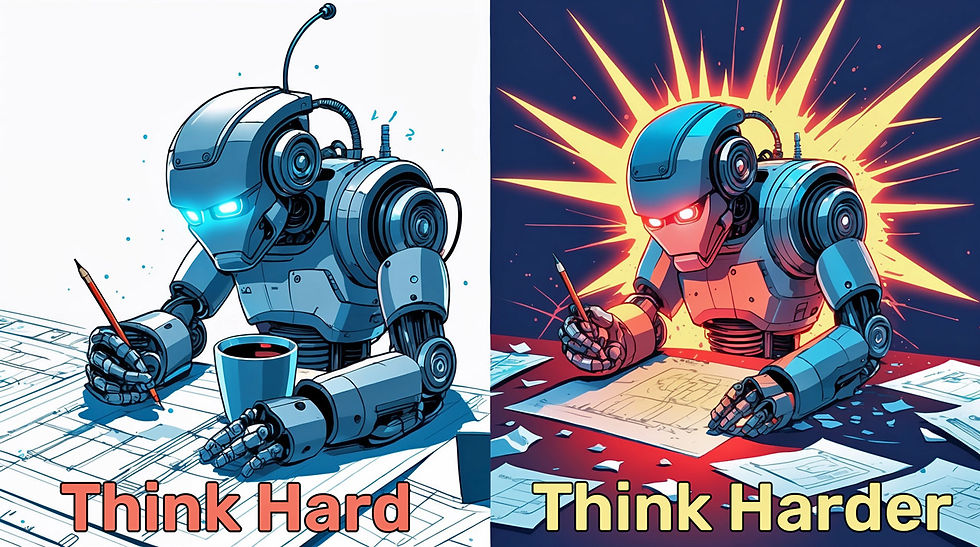
Thinking harder (spending more test-time compute) gives better results from AI reasoning models. (Leonardo)
AI Task Scope Doubles Every 7 Months
An interesting new type of AI scaling law was recently described by METR (Model Evaluation & Threat Research). They measured the scope of tasks that can and cannot be done with AI and also estimated how fast this scope has been expanding.
First, they defined a set of 170 tasks, ranging from small to large, that take between 1 minute and 30 hours to perform when done by humans. (In an approach dear to my old human factors heart, they observed experienced employees performing these tasks and measured how long they took.)
Second, they had various AI models attempt the tasks and scored them based on whether the tasks were completed correctly.
The first finding is that current cutting-edge AI, such as Claude Sonnet 3.7, has an almost 100% success rate on tasks that take humans less than 4 minutes but succeeds less than 10% of the time on tasks that take humans 4 hours.
Narrow-scope tasks: AI good. Medium-scope tasks: AI bad. Big-scope tasks: forget about asking AI.
But that’s now. The researchers repeated their study with AI models released since 2019. They found that the length of tasks that AI can do is doubling every 7 months. This is a super-fast scaling that beats old models of computer capability growth, such as Moore’s Law. METR’s report has many juicy charts supporting this conclusion: the fit between the data and the regression lines is almost uncannily good, with an R-square of 0.83 for the relationship between the logarithm of the human task time and AI’s success rate for that task. (R-square captures the amount of variance in one variable that is “explained” by the other. Thus, R2=.87 means that only 13% of the variance in AI performance is due to factors other than the task scope as measured by the time humans need to perform the task.)
I particularly like that this new measure of AI capabilities is related to human performance of real tasks and not on scoring well on math or physics tests like most other AI benchmarks.
Let’s use the estimate that the scope of successful AI tasks doubles every 7 months and consider 4-minute tasks as the current extent of AI’s capabilities as of February 2025, when Claude Sonnet 3.7 was released. This gives us the following estimates of what AI should be able to accomplish at the end of 2027 (when I expect AGI to be achieved) and 2030 (when I expect superintelligence):
December 2027 (year of AGI): 2 hours.
December 2030 (year of superintelligence): 68 hours = 8.5 working days.
Thus, even a superintelligent AI will probably not be able to perform the most demanding of human tasks that require several months of planning and execution. On the other hand, once AI becomes capable of routinely performing a task that takes humans 8.5 working days, it will do so in a few minutes.
How can I say that we’ll likely achieve superintelligence by 2030 if the prediction is that it will only be able to handle tasks of less than 8.5 working days? The answer is that human intelligence and AI are not equivalent. AI will be more intelligent than humans in performing short tasks, even if it can’t maintain planning focus over long periods of time. This means that the human role in the AI-human synergy that I continue to predict will be to have agency: to set strategic goals and monitor the AI. In contrast, AI will be the agent that will execute all those day-long and week-long tasks. Remember that a project that could last years is composed of many smaller tasks, often lasting less than a week. AI will perform those tasks, and it may even create the initial project plan for a year-long project. However, humans will direct the long-term progress of the project as these tasks are gradually completed.
Thus, the expected different focus of the two partners is:
Humans: Agency
AI: Agent
(These two words sound similar, but are almost opposite in terms of project responsibility.)

The human-AI synergy of the future will assign different responsibilities to the two partners, based on their relative strengths.
Also, we may not require AI to achieve close-to-perfect performance on a task, if we keep humans in the loop. For example, Sonnet 3.7, which can only complete 4-minute tasks to perfection, can currently perform 15-minute tasks with 80% accuracy. This means that if a human double-checks Sonnet’s output, the human will only have to correct 1/5 of the work. Assuming that it’s much faster to check work than it is to perform it in the first place, a company could still realize substantial time savings by assigning tasks around the 15-minute scope to an AI-human pair.

AI is rapidly improving the scope of tasks it can perform. In fact, this illustration could almost have been a screenshot from one of the many recent videos of robot demonstrations. (Midjourney)
Customer Journey Management
The design field has an equivalent to the task scope for AI discussed in the previous news item. We have lots of smaller-scope design tasks, such as designing a screen or a color palette. Many of these may currently take a human designer more than 15 minutes and thus be beyond assigning to an AI, even with human oversight to correct the 20% mistakes. But in a few years, this will no longer be the case.
Many individual design tasks will be done by AI by 2027, and most will be taken over by 2030. What’s the need for human UX professionals in this likely scenario?
Similar to what I just described, humans will retain the agency to manage design strategies over the broader scopes that still remain beyond AI’s capabilities. The largest strategic design scope we’re currently engaged in is customer journey management, which involves designing and optimizing customer interactions across all touchpoints and channels to create seamless, relevant experiences that drive the desired business outcomes. This idea goes beyond viewing customer interactions as isolated events, recognizing them as interconnected parts of a larger journey.
Journey management is a step beyond simply designing a specific customer journey for a particular user task, important as this is.
I am in the fortunate position to be able to recommend a consultant specializing in journey-centric design: Kim Flaherty from Resonant XD. I worked with Kim in my previous job, where she was my customer journey expert, so I know her talent and expertise well.
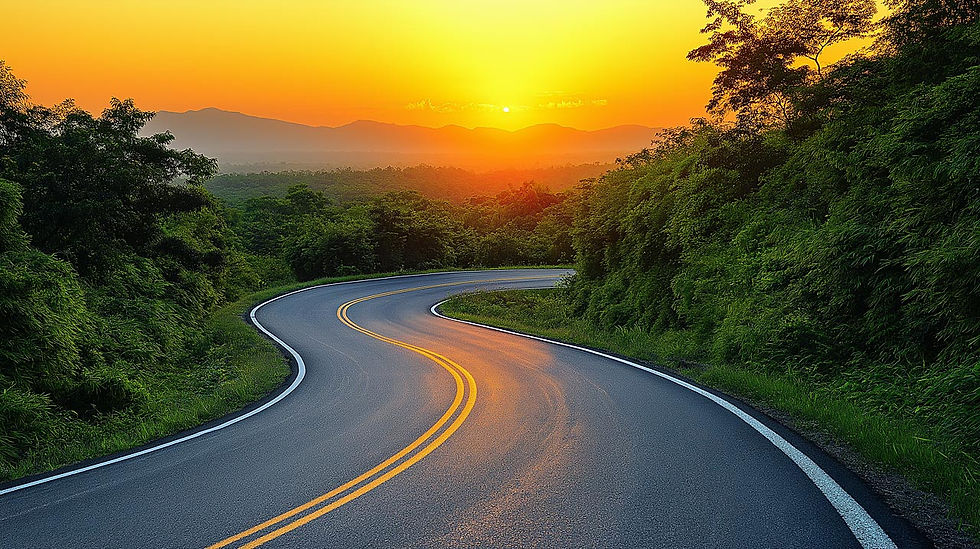
Steve Jobs’s classic saying, “The journey is the reward,” may be true for a road trip but not for most customer journeys. Truly mature design practices will recognize the need for journey-centric design on top of UI and UX designs, both of which remain important, but will increasingly be done by AI. The longer-scoped journey management is likely to be a human-driven endeavor for many years. (Midjourney)
3 New Image Models: Reve, ChatGPT Native Image Mode, and Ideogram 3
We have a new AI image tool, Reve, which launched at the top of the AI image leaderboard for prompt-adherence in image generation. (“Prompt adherence” basically means that it does as it’s told. As an example of poor prompt adherence from Midjourney, I asked for an image of a question mark next to an exclamation point, but got two question marks. Reve delivered the two symbols as requested.)
Here's an image I created with Reve to illustrate the point that, although the ROI of UX work has declinedsince the dot-com bubble, we can expect a revival over the next five years, as AI is uncovering new low-hanging fruit with the proliferation of poorly designed AI tools.
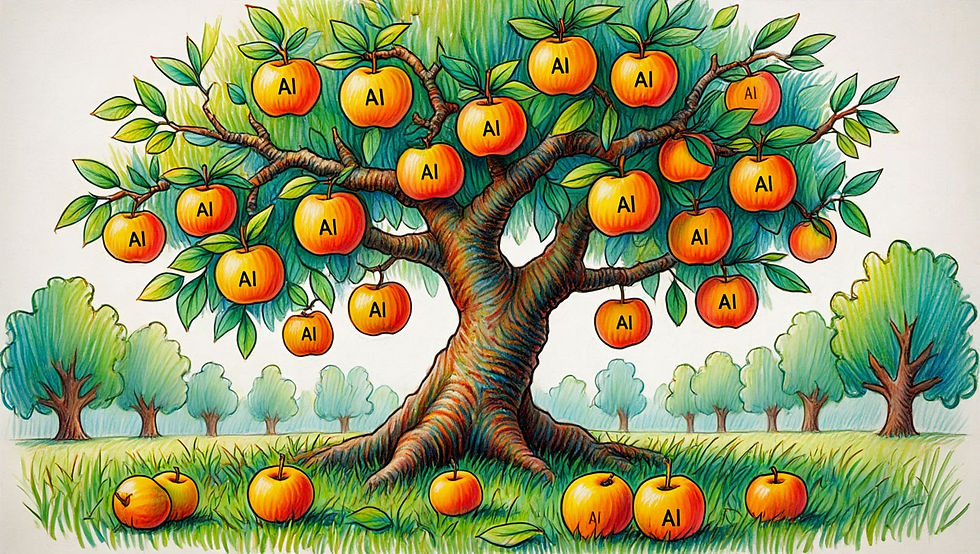
During the dot-com bubble, UX had sky-high ROI because the terrible usability of early websites created so much low-hanging fruit that could be discovered with a minimum of usability research and a small amount of design rework. The beginning of the AI era comes with a new tree full of low-hanging fruit for AI. (Reve)
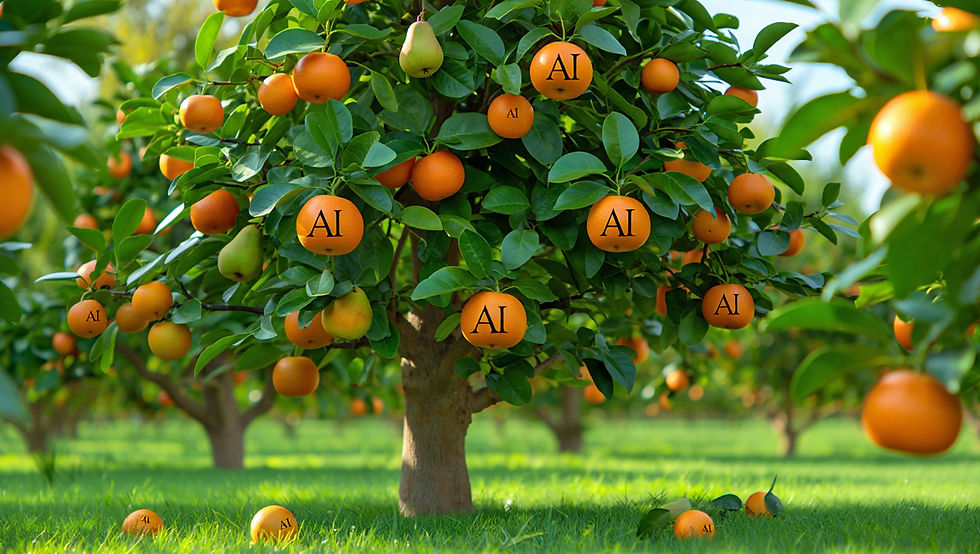
Reve’s first attempt with my prompt for a fruit tree with low-hanging fruit labeled “AI.” I didn’t like the aesthetics, so I used Reve’s “instruct” feature to tell it to change the image into a more painterly style.
As this example shows, Reve can change the style of an image based on a simple iterative prompt. I could also have changed specific image elements, for example, by saying “change the fruit into pears.”
I like Reve and will test it more once it graduates from preview mode. It is no surprise that Reve is good: I discovered that Luke Wroblewski is involved with Reve. He is one of the world’s leading UX designers.
What’s interesting about Reve is that it's a new type of AI image generator. Previous models have been primarily based on replicating image styles without a robust world model or language understanding, resulting in numerous examples of AI-generated images that don’t appear entirely genuine. Reve attempts to overcome these problems.
In other generative AI news last week, Ideogram launched its version 3, featuring significantly improved image quality, and OpenAI introduced a native image model for ChatGPT 4o to replace the aging Dall-E 3, which was becoming increasingly outdated compared to other AI image generators.
ChatGPT’s new image model may be even better than Reve at understanding natural language prompts and creating images that comply with the user’s intent. Here’s the image I got from the prompt: “Make a wide image in an illustrative style with elements of a satirical cartoon of a showdown between 3 AI image models: ChatGPT, Ideogram 3, and Reve. Label all 3 characters so that readers know who they are, and draw each one with some characteristic of their parent company.”
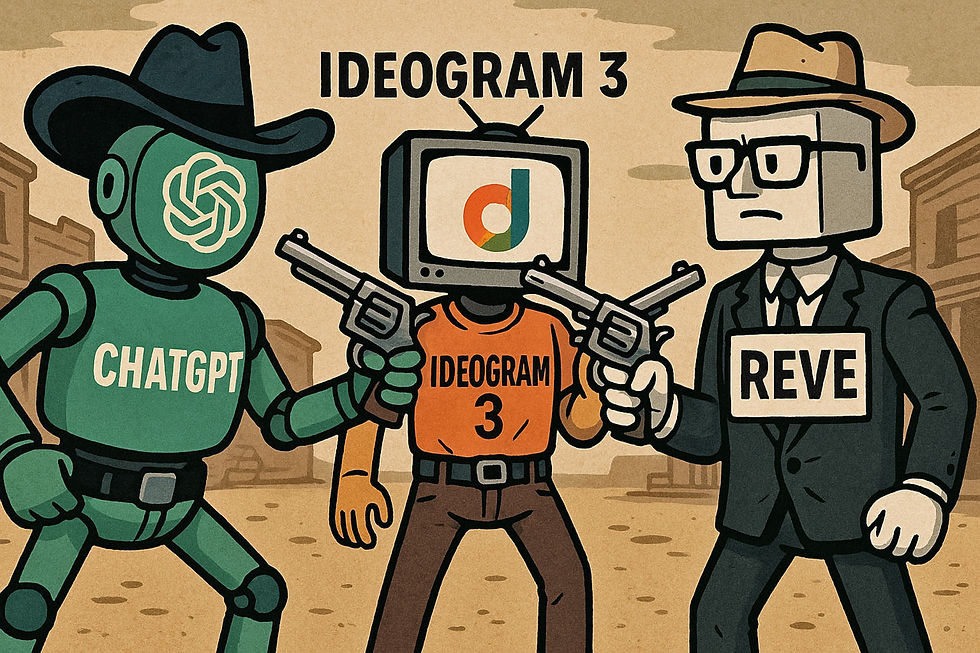
3 new AI image models were released last week. (ChatGPT 4o native model)
I then gave ChatGPT the following instructions for modifying the image: “Since this is an image-creation showdown, replace the guns with paintbrushes. Also, make the Reve character look French. Finally, change the logo displayed on the Ideogram character's monitor so that it looks more like Ideogram's logo.” Here’s the result:

ChatGPT still didn’t get Ideogram’s logo right, but it easily swapped the guns for paintbrushes and gave Reve that stereotypical French look. (I don’t know how tightly connected Reve is to France, but the name certainly sounds French.)
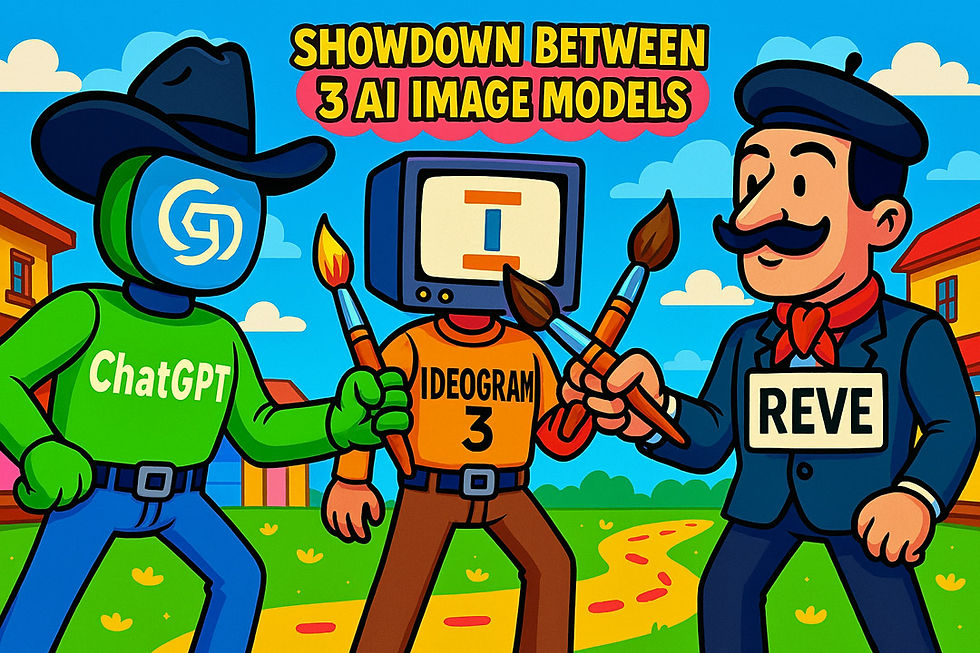
My final image-modification prompt was: “Make the cartoon more funny and colorful.” Brighter colors? Yes. Funnier? No. We still need human agency for better storytelling, even when using generative AI. Interestingly, OpenAI’s own logo was only rendered correctly in the first version and then disintegrated in the redraws. (ChatGPT 4o native model)
Practical tip: ChatGPT 4.5 is better for generating words, and ChatGPT 4o is better at generating images. I am unsure whether the absence of the new image model in GPT 4.5 is a temporary limitation due to the rollout of the update. It’s an obvious usability problem that users must know such esoteric AI lore and select the correct model for each task. OpenAI will reportedly unify all its models with the forthcoming GPT 5 upgrade, so perhaps they aren’t highly motivated to address UX flaws in their legacy models.
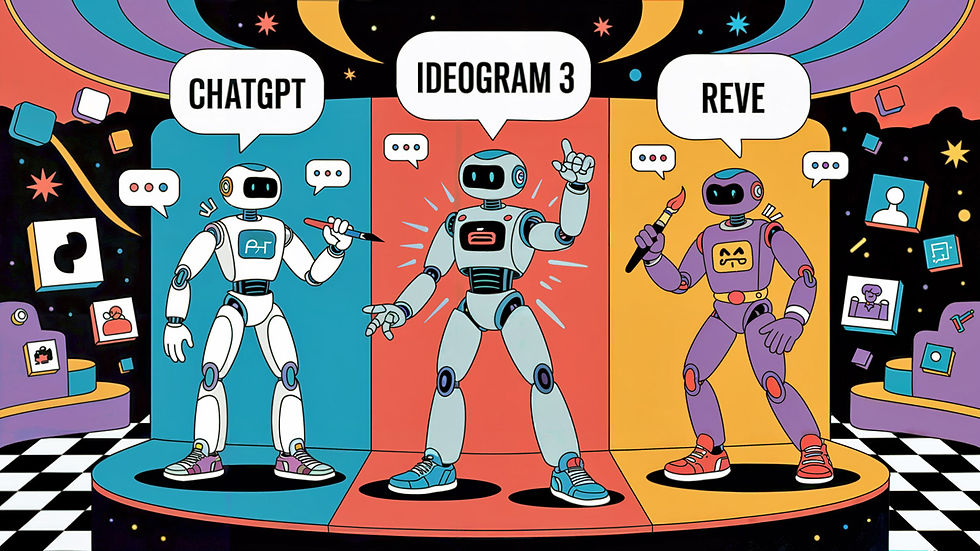
Showdown between the 3 advanced image models, as drawn by Ideogram 3. I don’t think this is as engaging as the images produced by ChatGPT 4o’s native image model. ChatGPT benefits from integrating image generation with a beefy general-purpose AI that can think up more interesting scenarios.
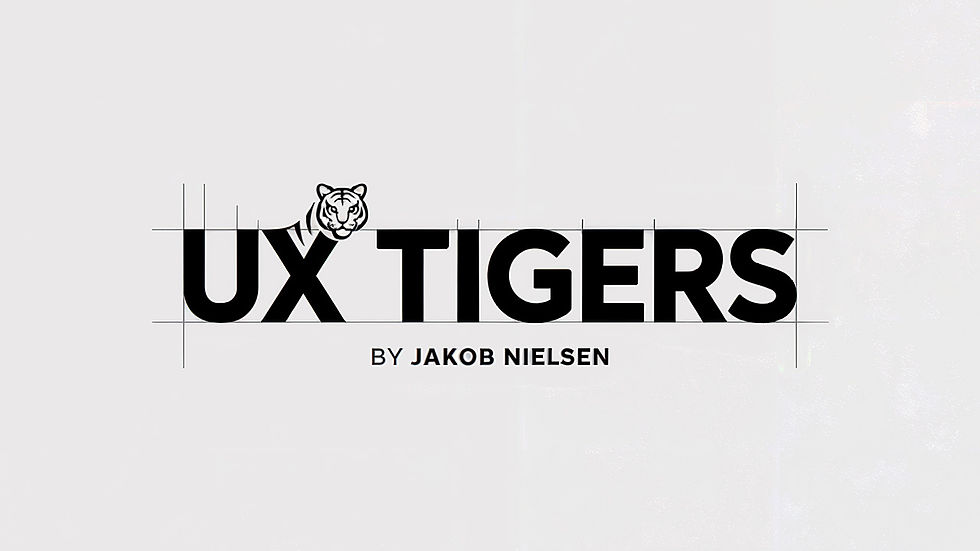
Here is Ideogram 3’s proposed logo for my website. I'm not sure why it inserted some gray inside the letters of my name. I am sure that any good visual designer will deplore this as the equivalent of beginner logo design, but the point is that now we can obtain such images, and they are spelled correctly. Next release, they’ll be better. For now, it’s the Fiverr logo designers who’re in trouble. In five years?