Summary: Cookie banners waste 575 M hours of European users’ time annually | Jensen Huang on AI infrastructure | AI training clusters keep growing | 2 minutes summary of 59-minute keynote | Virtual try-on reaches the masses
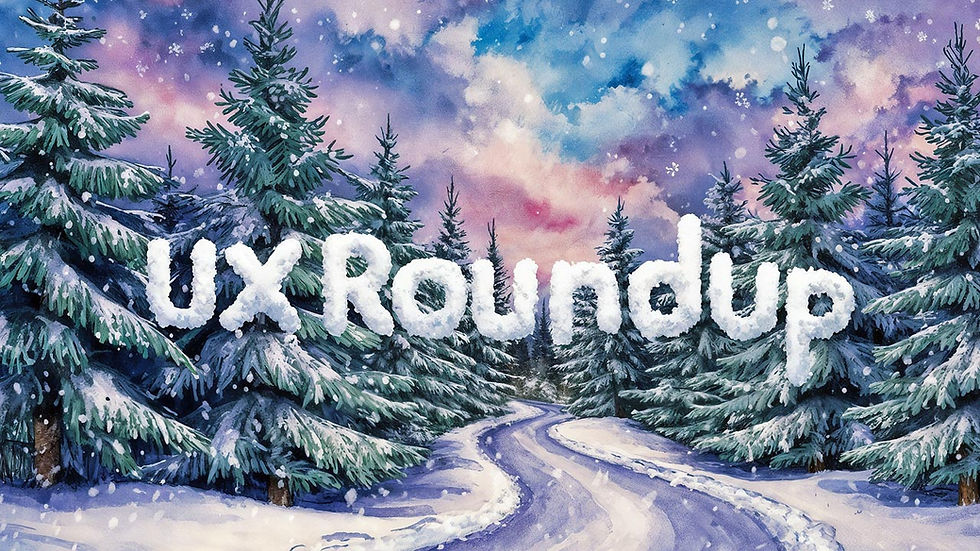
UX Roundup for December 2, 2024. Northern hemisphere winter is on its way. (Ideogram).
Cookie Banners Waste 575 M Hours of European Users’ Time Annually
An analysis finds that the infamous GDPR cookie-consent banners waste 575 million hours per year for users within the European Union. Since these banners also plague users outside the EU, the worldwide cost is even higher.
In the EU alone, users' time on clicking useless cookie banners equates to €14 billion (USD $15 B) per year in productivity losses at average European wages.
These banners truly are useless, because a large-scale analytics study found that only 0.1% of users use the potential benefit offered by the banners to review the cookies and save their preferences for which cookies to accept. 5% of users do reject all cookies, but that choice is better implemented as a browser setting which would be applied automatically without delaying the user.
Cookie banners are terrible for usability. Not just because they destroy half a billion hours of human lifespan without any benefit but also because they are part of the general trend toward information pollution. The more extraneous information you throw at users, the less they are capable of paying attention to the things that really matter.
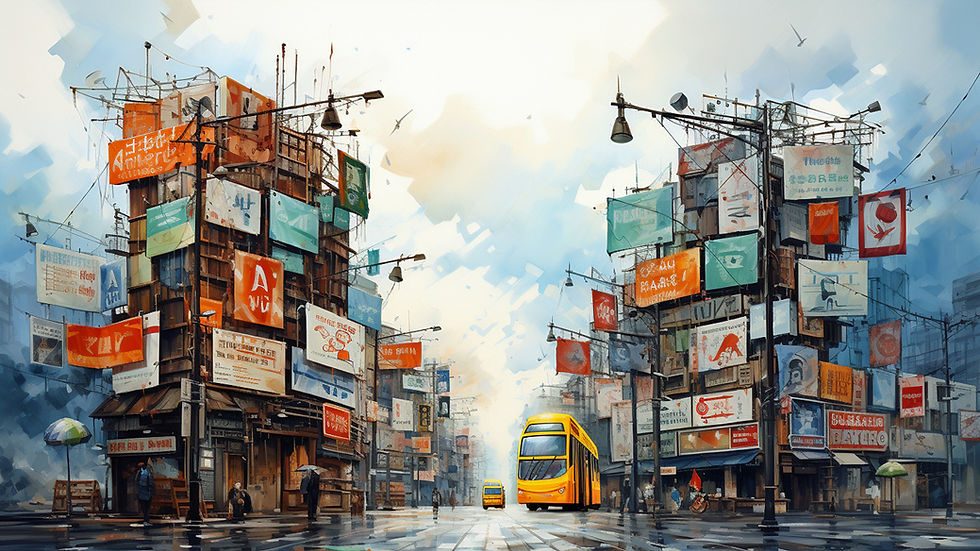
Too many banners and popups pollute our information environment. (Midjourney)
More banners and popups equal more popup hate, which again causes users to dismiss any such notifications, even ones that might have been useful to them.
Cookie banners are a case of the classic story of “crying wolf.” 99% of the time, the banners warn against something harmless, causing users to tune them out. Then, when users encounter genuine dark design patterns, they are defenseless and “agree” to “opt-in” to something they don’t want.
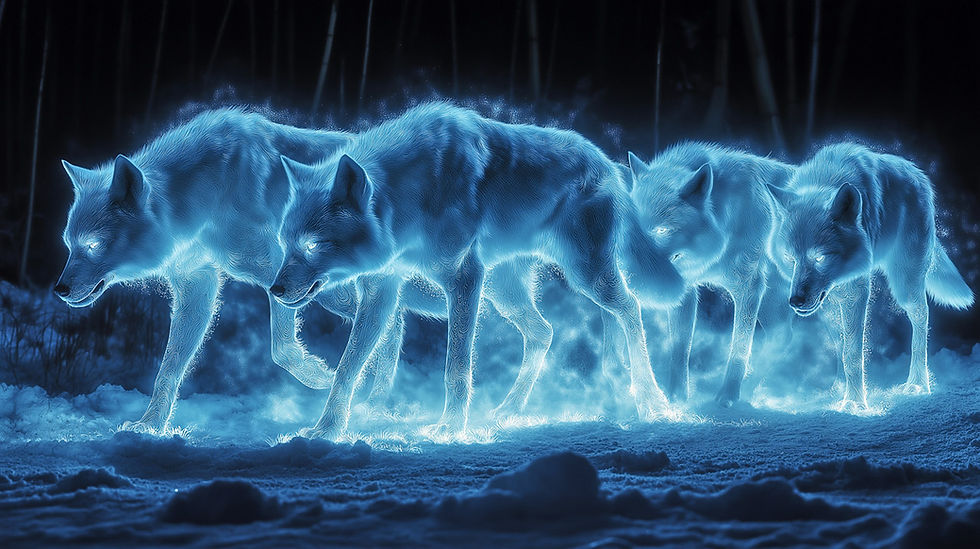
If you cry wolf too often, people will ignore you. But one night, a pack of wolves may emerge from the snow. (Midjourney)
While cookie privacy is a non-issue, true privacy violations are a major problem on the Internet. However, the way to fight privacy violations is not through naïve GDPR regulations and user interface design by EU bureaucrats. Instead, cancel all the rules causing cookie-banners and spend less than 10% of the saved €14 billion on funding a privacy-enforcement agency with genuine investigative powers to after the companies that commit true privacy violations, such as spam or false opt-in driven by dark design.
I would favor such an agency going easy on first-time offenders, especially small companies who might not know any better. Such cases should be resolved with a warning. But repeat offenders should be severely prosecuted. Again, get rid of the pop-ups, and you have €1 B to handle the worst cases aggressively.

The cookie cops should be friendly to first-time offenders but aggressively investigate and severely prosecute repeat dark pattern abusers of users’ privacy. (Midjourney)
Jensen Huang on Building AI Infrastructure
Jensen Huang (head of NVIDIA) gave a great interview to the “No Priors” podcast (YouTube, 37 min.). I admit that I used to think of Huang as a hardware guy who was not that relevant for my thinking. However, he impressed me with his visionary thinking that goes far beyond building GPU chips. (It also helps that Huang is mega-charismatic. Compare Huang’s blazing bonfire of visionary thinking with a recent interview with Sam Altman, who has the charisma of a 15-watt lightbulb.)
Huang stated that NVIDIA believes it can double or triple the AI compute performance of its systems every year. This is 4 to 6 times faster than the traditional Moore’s Law for algorithmic compute, which doubles every two years.
Why can AI compute scale faster than traditional CPU compute? Because NVIDIA takes a whole-system approach to designing its hardware. They don’t just build the same hardware with ever-denser semiconductors. They redesign the GPUs and other interconnected components with a view toward optimizing the performance of the entire training cluster.
As a result, this “hyper Moore’s Law” can be expected to produce 10 doublings or triplings over a decade, cumulating to gains of 1 K on the low end and 59K on the high end. Let’s take an average and expect a 10-year improvement in raw compute of a factor of 30 K.

Fast (the traditional Moore’s Law) vs. faster (the “hyper Moore’s Law” targeted by NVIDIA). (Midjourney)
According to the AI scaling law, 10 years correspond to about 3 generations of AI improvements. Each generation requires about 100 times the effective compute of the previous one. 3 generations will therefore require a million times more compute. The AI generation being trained now uses 100,000 Hopper GPUs in the case of xAI, which has published the size of the training cluster it uses to train Grok 3.
With no improvements in the hardware, we would thus need a hundred billion chips to train the super-superintelligent AI we hope to get around 2034. (Regular superintelligence is expected around 2030, but I think we can go to super-super, meaning dramatically smarter than the highest-IQ human who ever lived.) It seems infeasible to build a datacenter with 100 billion GPUs. Luckily, if NVIDIA delivers even on the low end of its promise, that cuts the compute need to 100 million GPUs. Very hard to build, but not impossible.
However, the scaling law talks about effective compute, not raw compute. Effective compute includes the benefits of algorithmic efficiency — the ability for smarter programming to require fewer compute cycles to achieve the same result. Current AI software is horribly ineffective since it was constructed on the fly by developers who basically don’t know what they are doing: they’re just experimenting. (All honor to that: those supergeeks built AI that works, which people like myself never thought would happen.)
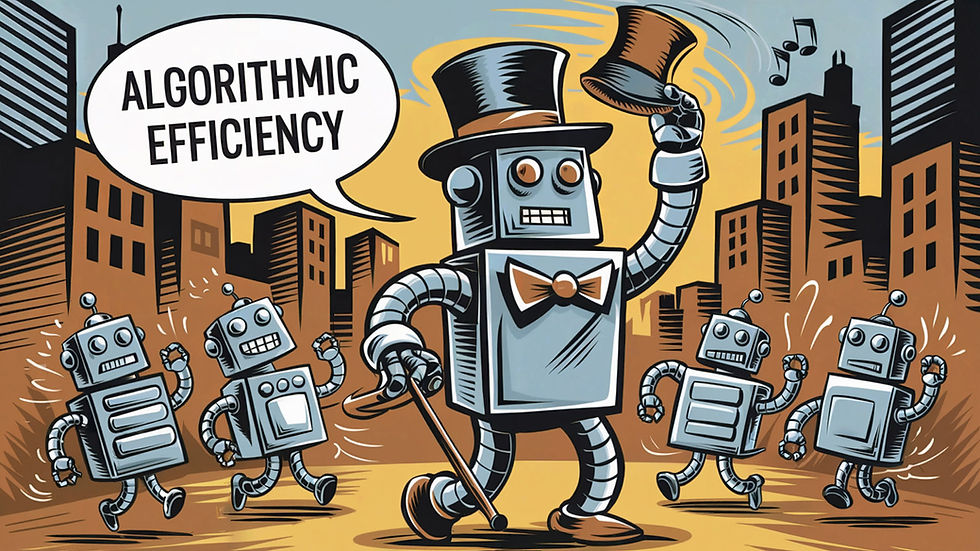
Algorithmic efficiency will make AI dance. (Ideogram)
How much algorithmic efficiency can we expect over the next decade? I’m bullish, especially for the years after 2027, when we expect Ph.D.-level AI that will be a better programmer than almost any human. (And after 2030, when AI will be a better programmer than any human.) Not only will AI be a good programmer, but we can unleash millions of AI programmers to explore many programming ideas that are either too complex for any human brain to envision or too unlikely to work to be worth experimenting with as long as we only have a few thousand human programmers working in each AI lab.
I would not be surprised if the next decade gives us a factor of 100 improvement in algorithmic efficiency. This gives us a final estimate that a frontier AI training cluster in 2034 will contain a million GPUs. Seems highly feasible, given that xAI has already announced that they’re doubling their current training cluster from 100K to 200K GPUs in 2025. A further factor of 5 in 9 years should be possible.
Another exciting point in Jensen Huang’s interview is that he disclosed that NVIDIA already uses AI to design their GPUs. Human designers cannot envision the full complexity of chips like Blackwell, let alone future generations. Thus, if NVIDIA had to rely on human designers, improvements could only happen on the scale of small subsystems of the entire GPU. Senior designers would then cobble these subsystems together to form the final product, but this is a seriously suboptimal design process compared to designing the full system as a whole. Only AI is capable of such system-scale design.
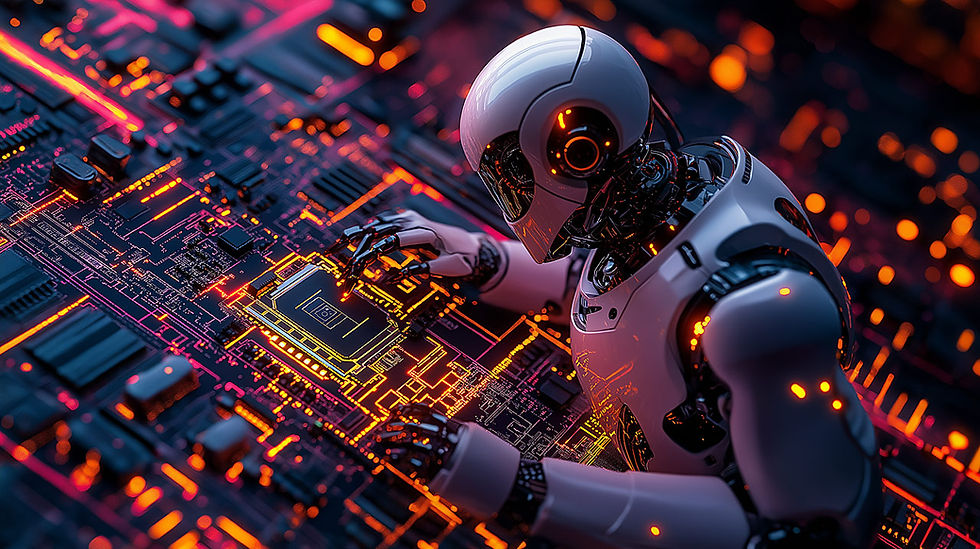
AI is already designing the hardware that will be used to train the next generation of AI models. These systems are too complex for human designers to grasp fully. (Midjourney)
While Huang didn’t mention this, I think a similar phenomenon applies to user experience design. Big user interfaces, such as Amazon.com or the Office-Windows combo at Microsoft are too big for any human designer to understand fully. (Or for any human researcher to study in depth.) Thus, UX designers and researchers work on a subsystem level and are incapable of designing the total user experience that we’ve always held out as the goal.
I’m hopeful that AI-based UX research and design will recapture our ability to design large systems as a whole, with the resulting usability improvements.
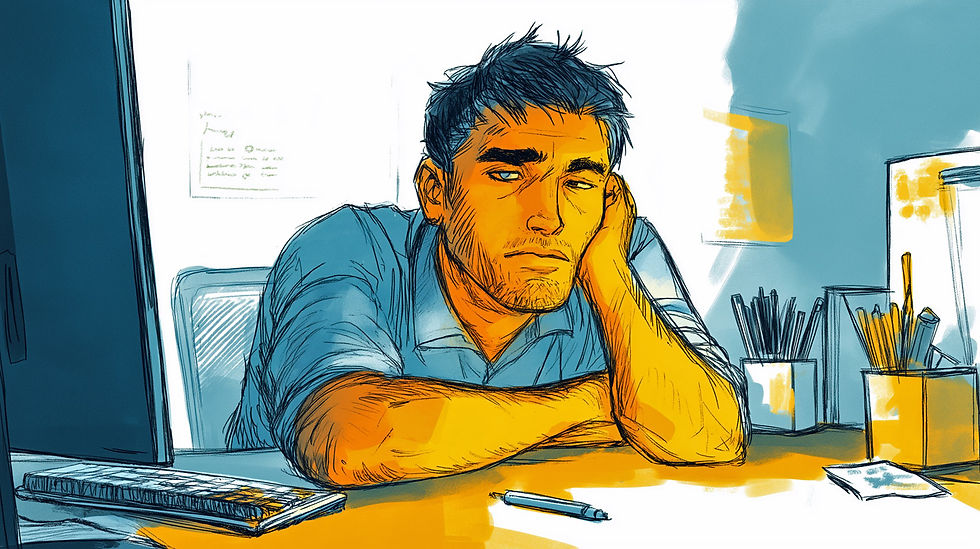
Poor human designers may be unable to understand the full system beyond a certain level of complexity. Hopefully, a synergy between AI’s large-scale brain (with context windows 100x the current max) and humans’ intent-driven urgency will perform better. (Midjourney)
Bigger Is Better
I just mentioned that xAI has a training cluster of 100K GPUs for Grok 3. It’s the one AI lab that’s forthcoming with details about its datacenter. (You can even watch a 15-minute walkthrough video of the xAI training cluster.)
Not wanting to be left behind, Mark Zuckerberg recently announced that the Meta training cluster now has more than 100K GPUs. (He didn’t specify the exact number, so in principle, it could just be 100,001 GPUs.)
Of course, this announcement caused xAI to go public with its plans to double its training cluster to 200K GPUs in 2025. These competing announcements made several Internet wags complain that bragging “mine is bigger than yours” is what counts for innovation in AI these days.
However, it is unfair to denigrate the research efforts needed to build a new world record datacenter with 200K GPUs just a year after the feat of making one with 100 GPUs which had also never been done before. Just because datacenter engineering is not my field, to say the least, doesn’t mean I don’t recognize the challenge.
I remember when I was a Sun Microsystems Distinguished Engineer. This 30-person team of 28 supergeeks and 2 UX guys (me and Bruce “Tog” Tognazzini) comprised the company’s 0.1% top technical talent, so I had the privilege of working with some of the world’s most elite hardware and software engineers. I remember the hardware guys telling stories of when they invented a 100x better component: it did almost nothing for the performance of the beefy servers they were building. They had to figure out how to improve everything, such that the entire architecture functioned at that 100x accelerated level.
Ever since I’ve deeply respected the hardware people who create the infrastructure the rest of us rely on.
For AI training, bigger is better, but size doesn’t come from writing a big check to purchase more CPUs. It requires seriously cutting-edge engineering innovation.

Bigger is better. More compute creates smarter AI. (Ideogram)
AI Avatar Summarizes Keynote Talk
I made a new AI avatar video to summarize my recent keynote presentation at the Y Oslo conference. The full video of my talk runs 59 minutes: I think it’s worth watching if you have an hour to spare.
If you don’t have time for the full thing, that’s what the 2-minute avatar summary is for.
I made this new avatar video with the specialized AI-avatar creation service D-ID, using its image-to-video capability. First, I used Midjourney to make the base image of “a Norwegian TV presenter” (since this video was for reporting on an event in Oslo, Norway).
D-ID has a huge library of fairly good voices, so I am reasonably pleased with the soundtrack for this avatar video. I picked a European accent for added verisimilitude.
I then made a few other animations with Kling for visual variety in the final video and added subtitles in CapCut. (I also used CapCut to format the avatar video in two aspect ratios: 16:9 widescreen for YouTube and 3:4 portrait format for social media.)
Compared with the previous avatar video I made with Humva, I don’t like the lip-synch animation of D-ID’s image-to-video avatar. It has a nasty “uncanny valley” feel.
D-ID does offer great usability for avatar creation: very easy to use, and you don’t actually need to create your own base image, because they have many stock avatars available.
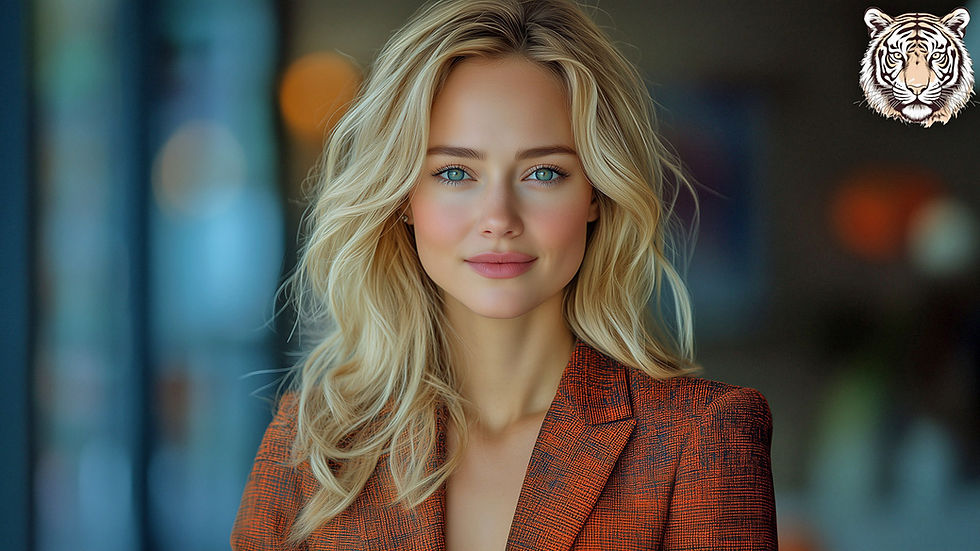
This “Norwegian TV presenter” was the basis for my new D-ID video avatar. (Midjourney)
Despite my complaints about lip-synch fidelity, I prefer this avatar video as a summary of my keynote, compared with the AI-generated podcast summary I made earlier with NotebookLM.
The avatar video only runs 2 minutes, whereas the podcast consumes 9 minutes of viewers’ time. The information density is much higher in the version that emulates a newscast instead of a podcast. Admittedly, the podcast format is more entertaining, with the back-and-forth between the two hosts.
Which format do you prefer, as a way of summarizing longer content? Let me know in the comments.
Norwegian AI company Breyta has produced an interactive space about the Y Oslo conference where you can ask questions about the talks, including my keynote (and several of my articles). This is an exciting way to reuse the extensive, but fleeting, content from a conference in an interactive, durable manner.

Breyta’s tool transforms a series of conference recordings into a living environment that can be queried for insights. The main use case for their product is to glean conclusions from recorded user research sessions. (Ideogram)
Virtual Try-On Reaches the Masses
Virtual try-on is using AI to create images and videos of any model (including yourself) wearing any outfit without a photoshoot.
In mid-2023, this feature was one of my predictions for future AI-driven enhancement of e-commerce sites selling fashion. It’s always tough to envision whether an outfit will look good on you simply from seeing it on a professional model.
By late 2023, Google had launched virtual try-on as a feature, presumably targeted at their deep-pocketed big customers.
Virtual try-on remains a promising feature of generative UI that can be used to create an individualized user experience for shoppers and, thus, presumably increase sales. (And decrease returns.)
Now, we’ve progressed one step further toward realizing this vision: The AI video generator Kling launched an “AI Try-On” feature last week, allowing anybody to produce custom videos with any outfit. Kling subscriptions are $10 per month: progress in AI is making formerly-advanced features available cheaply in a year. The moat is drying out.
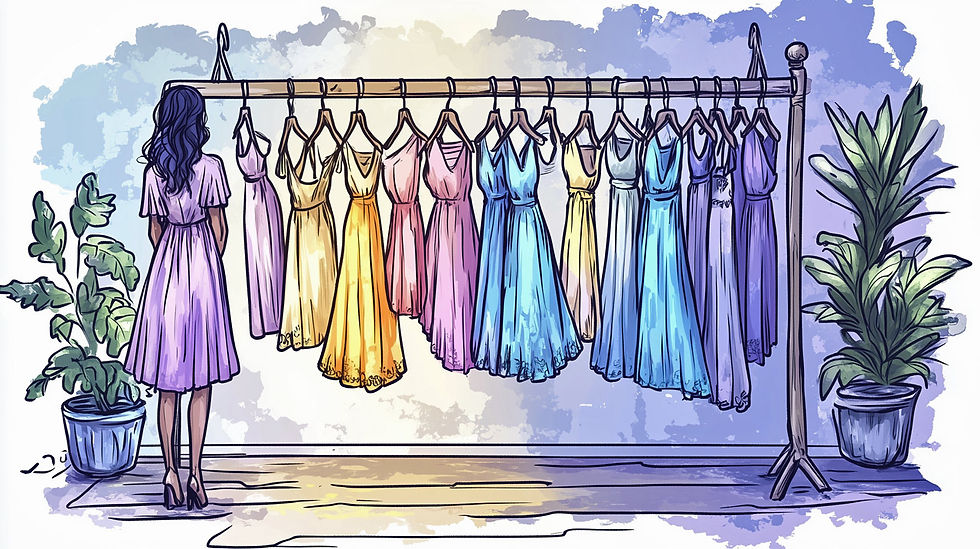
No end of outfits to try on. One click, and Kling makes a video of the same model with that new outfit. (Midjourney)