Summary: Consultants don’t work for improving productivity through AI | Apple AI = bad AI | AI is a general-purpose technology | Generative UI for the enterprise: Salesforce’s AI-designed dashboards
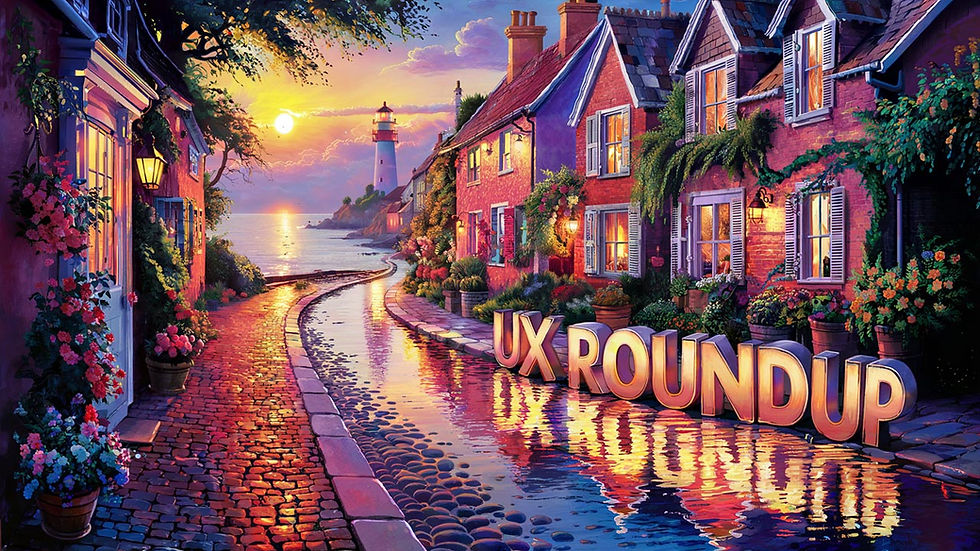
UX Roundup for October 10, 2024. (Ideogram)
Consultants Don’t Work for Improving Productivity Through AI
I ran a UX consulting company for many years, and I still feel the shame from charging clients for projects they would have benefited from doing themselves. (Possibly with coaching from an expert, but it proved impossible to bill clients for coaching.)
Ethan Mollick is probably the world’s leading expert on using AI in business. He recently published a perceptive newsletter on tactics for improving AI use in organizations. Mollick starts by reviewing the paradox that, on the one hand, many business professionals use AI, and this use is shown in controlled studies to improve productivity by around 25-40%. (More for programmers.) But on the other hand, business executives say that they see little AI use or productivity gains from AI in their companies.
Mollick’s explanation is that individual use and organizational use are different. He says that “to get organizational gains requires R&D into AI use.” And here’s the kicker: each company has to do this research itself. For AI use, you cannot outsource organizational innovation to consultants or enterprise software vendors who develop generalized approaches based on what they see across many organizations. It’s questionable how well this method worked for traditional technologies, but it definitely doesn’t work for AI.
The point is that nobody knows yet how to best use AI in specific industries or specific companies. This is even more true considering that we will change to a completely different generation of AI capabilities in about 3-4 months. Once we get models based on 10x more training, their skill level will change from that of a smart high school graduate (the GPT 4 generation of AI we’ve been using since 2023) to that of a college graduate (the GPT 5 generation that is expected to launch soon).
The way to discover productive use cases for a given company is for that company’s own employees to experiment heavily with AI for their own work processes. As Mollick says, “Experimentation is hard and expensive for outsiders trying to develop new products, but very cheap for workers doing their own tasks.”
Many people are indeed conducting such experiments with personal use of AI, either to safeguard their careers (you need to be an AI expert to have a job in a few years), or to make it easier to complete their assignments. But crucially, people are not sharing their findings with company management. Most companies don’t support or reward employee experimentation, outside of official research groups. On the contrary, many companies have harmful policies about the use of “unauthorized” technology, like AI. So, people stay quiet about their AI discoveries, to the detriment of the company’s future.
In contrast, we need to mix and match the discoveries of these individual employees to arrive at group-level synergies and the true productivity gains from AI.
Companies should give out big bonuses to employees who discover good AI uses for their work, and then publish these discoveries for others to use and develop further. Companies should also sponsor AI hackathons for each department to focus on specific use cases.
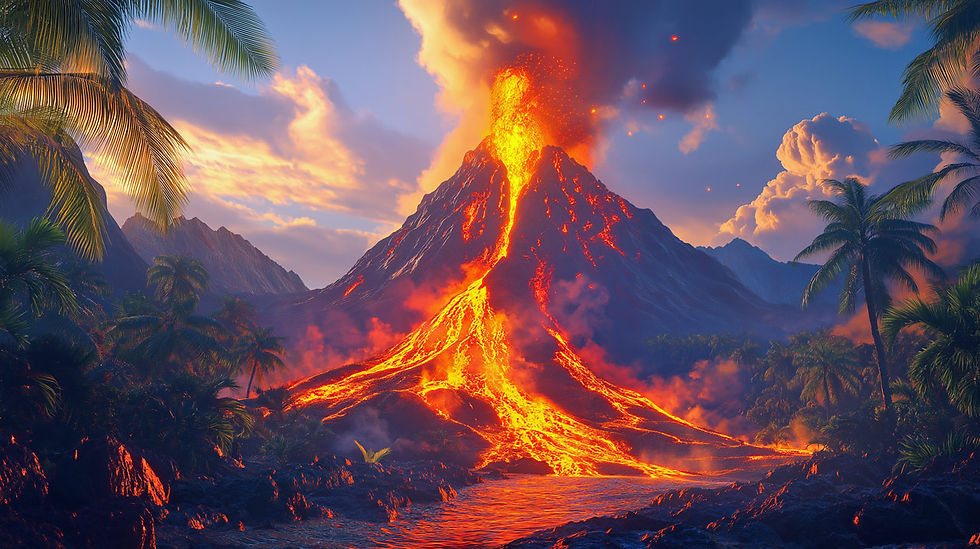
A volcano can serve as a metaphor for the best way for companies to discover profitable use of AI. You start inside the volcano, at ground level, where the “magma” builds up through grassroots experimentation. Workaday employees try myriad ways of using AI for their work, and soon enough, many will work, resulting in higher pressure. Eventually, we get an eruption of AI creativity, and the best AI-augmented workflows flow down the side of the volcano to reach those employees who didn’t experiment. The point it to start from the inside: don’t expect outsiders to save you with expensive consulting advice, because nobody knows the best way to use AI in any given business. (Midjourney)
Apple AI = Bad AI
The so-called “Apple Intelligence” is expected to launch for iPhone users any day. Ethan Mollick points out that its level of intelligence is roughly the same as GPT-3.5, which was the “baby AI” from 2022 that preceded the first high-quality AI (GPT-4 in 2023).
This as we are just a few months away from the release of the next generation of AI in December 2024 or early 2025. Whereas current AI is as good as a smart high school graduate, the next generation of AI is expected to be the equivalent of a college graduate. In two years, AI advances about the same as humans do in four years!
(In contrast, the “baby AI” of 2022 was closer to a smart middle-schooler.)
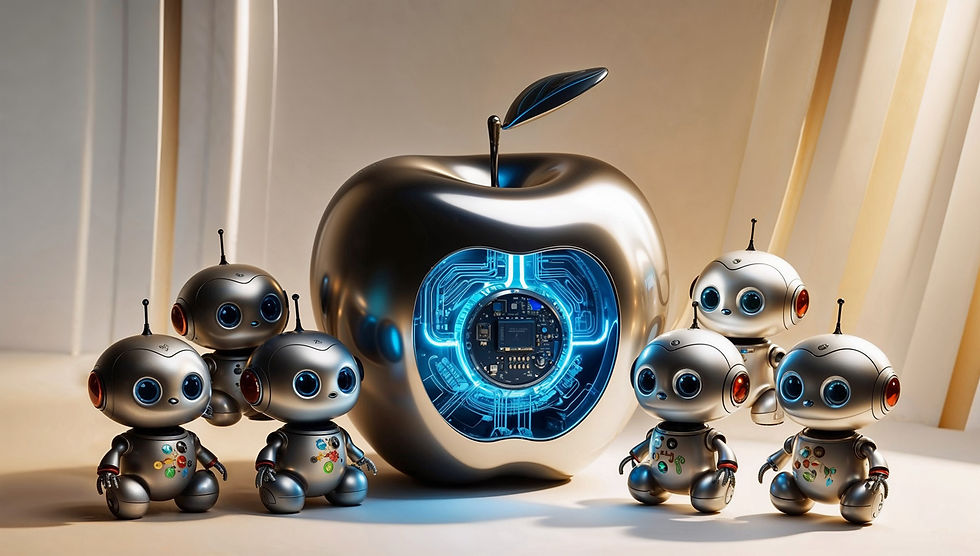
Apple’s forthcoming on-phone AI will be equivalent to the “baby AI” level of intelligence from the previous generation of AI (GPT 3.5 level), whereas the world will move to the next generation in a few months (GPT 5 level). Squeezing AI onto a phone is an engineering marvel but will give users the wrong impression of AI’s impact on their career and business. (Leonardo)
We should credit Apple with an immense engineering accomplishment in being able to squeeze a decent AI onto a phone. Much as I’m denigrating the intelligence of the previous generation of AI, it required a huge data center to run those models as recently as 2022.
However, as Mollick mentions, there is a big risk that many people will get their first hands-on experience with AI from using those middling “Apple Intelligence” features on their phone. Particularly by 2025, this will give them a completely unrealistic impression of cutting-edge AI capabilities. This again will make them midjudge the need for urgent career pivots and (in case they are company executives) for companies to embrace high-end AI and not just low-end AI.
This mismatch is that much more serious when you consider that humans move slowly, even as AI advances fast. Another two years or so (around 2027) will get us AI with capabilities similar to a large crew of Ph.D.s with doctorates in all scientific disciplines and specialties.
If somebody starts to pivot their career or company now, it could easily take two years for an “AI-first” mindset to come to fruition. This means that you should plan now for that Ph.D. level of AI< which will be almost impossible if your personal hands-on experience is with the middle-school level AI running on your phone.
AI Is a General-Purpose Technology (GPT!)
In the computer field, we’ve become accustomed to using the abbreviation “GPT” (as in “ChatGPT”) to stand for “Generative Pre-trained Transformer,” which is the technology behind most current AI models.
However, that exact same acronym has long been used in economics to stand for “General Purpose Technology,” which is an important concept in understanding how innovation impacts the economy.
General Purpose Technologies are characterized by three key features:
Pervasiveness: They have applications across many sectors of the economy.
Improvement over time: They tend to become more efficient and cost-effective as they develop.
Innovation spawning: They facilitate the creation of new products, processes, and even industries.
Several technologies throughout history have been identified as GPTs due to their profound and wide-ranging economic impacts:
The Steam Engine: This 18th-century invention revolutionized manufacturing, transportation, and agriculture. It powered factories, locomotives, and steamships, fundamentally changing the nature of work and trade.
Electricity: The widespread adoption of electrical power in the late 19th and early 20th centuries transformed both industry and domestic life. It enabled new manufacturing processes, lighting, and a multitude of electrical appliances.
The Internal Combustion Engine: This technology reshaped transportation, logistics, and urban development. It led to the creation of the automotive industry and dramatically altered personal mobility.
Information and Communication Technologies (ICT): This broad category includes computers, the Internet, and mobile devices. ICT has revolutionized information processing and communication and spawned entirely new industries.
AI now joins that list as a general-purpose technology.
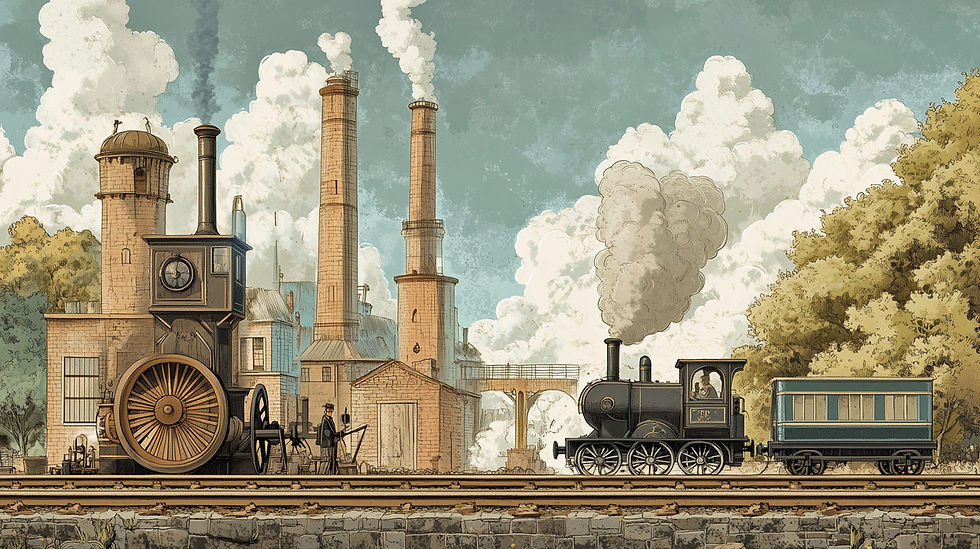
The original steam engines were stationary and used to drive factory equipment. In 1804, Richard Trevithick, a British mining engineer, had the brilliant idea to put a steam engine on wheels and tracks: the railroad came as a complimentary innovation. It took a few more years of additional inventions before George Stephenson’s Locomotion No. 1 became the first steam locomotive to haul passengers on a public railway in 1825. (Midjourney)
The introduction of a GPT often follows a pattern in terms of its economic impact:
Initial Slowdown: There’s often a productivity paradox where the initial adoption of a GPT may lead to a temporary slowdown in productivity growth as the economy adjusts.
Complementary Innovations: Over time, the GPT spawns complementary innovations and industries, leading to widespread productivity gains.
Long-term Growth: Eventually, the GPT contributes significantly to long-term economic growth, often reshaping entire economic structures.
Computers just recently moved into stage 3, after much time spent with low-usability systems that didn’t improve productivity as much as promised. Today, though, the economy would suffer without computers and communication technology.
AI is currently in phase 1: billions of dollars are being invested in compute clusters to train the next generation of higher-intelligence AI models, and yet companies don’t have many examples of firm-level productivity gains. (Much as we have plenty of examples of employee-level productivity gains for individual users.)
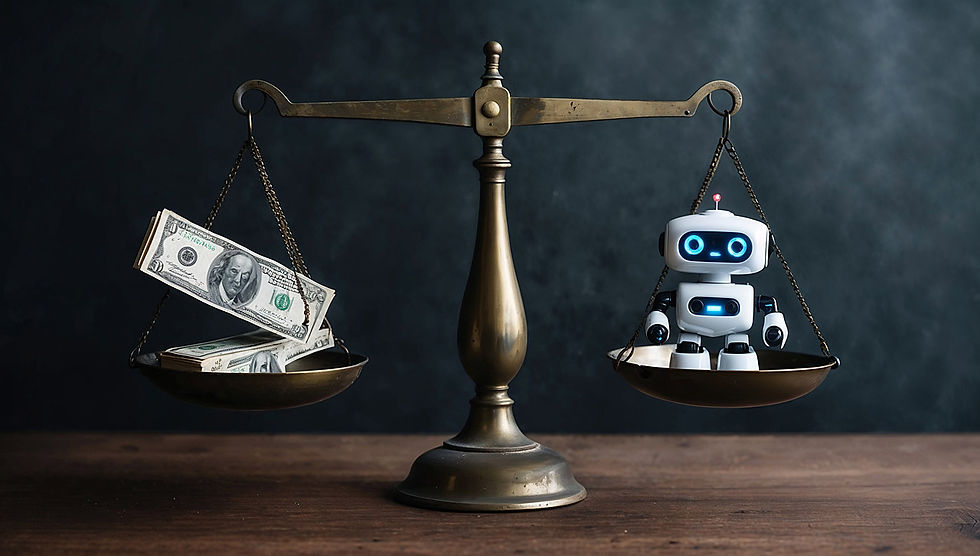
The cost-benefit tradeoff for AI is still in stage 1, where investments are large and payoffs are small. History suggests that this balance will change as we discover the best ways of using this general-purpose technology (see the first item). (Leonardo)
This entire issue was discussed in great detail in a recent lecture by Erik Brynjolfsson who is the world’s leading expert on the impact of technology on the economy (YouTube, 59 minutes). Well worth watching. In addition to being a Big Thinker, Brynjolfsson is also a good speaker, which is a rare combination. Well worth watching.
Generative UI for the Enterprise: Salesforce’s AI-Designed Dashboards
Generative UI (GenUI) is the technology for having AI design a user interface. Either as part of a traditional UX design process, or in real-time optimized for each user’s needs.
Enterprise products have long suffered from poor usability: they are complex and users’ needs differ. Salesforce is now introducing a new GenUI product called “Generative Canvas for Lightning UX.” This product will design an individualized dashboard for each user with the data and visualizations he or she needs for a current task.
Right now, this is only available in limited beta release for select customers. I don’t know how good it is or the usability of the AI-generated dashboard designs. However, this is a promising use case for AI in the enterprise, since the underlying data is already captured in the Salesforce system and can be made available to users in better ways than traditional one-size-fits-all dashboards.
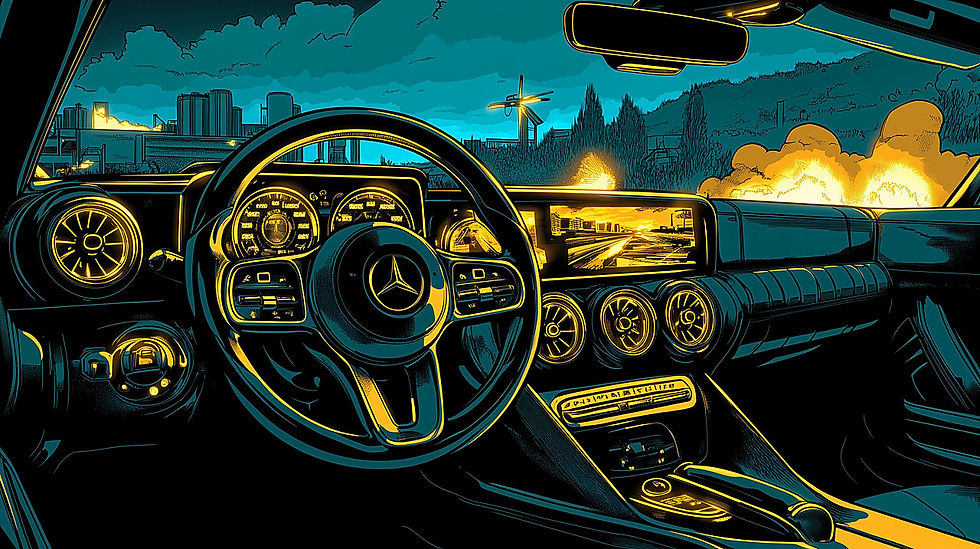
Dashboards are a metaphor from automobiles. In a car, you are restricted to the manufacturer’s design, even though computer screens give some flexibility. For enterprise users, we can now have a custom dashboard designed by Generative UI based on each user’s specific decision support needs. (Midjourney)
Comments