Summary: Recent studies reveal persistent human skepticism toward AI outputs despite superior performance in medical, creative, and analytical tasks. Human–AI collaboration succeeds when roles align with complementary strengths (AI: speed/scale; humans: nuance/strategy). Trust calibration through transparency, task-specific education, and bias-aware design emerges as critical for adoption.
What if your best employee was an algorithm you refuse to promote? Studies prove we downgrade AI’s work by default, even when it outperforms humans. Is this bias protectionism for our species… or just fear of becoming spectators in our own professions?
I have discussed many controlled research studies in this newsletter that have demonstrated immense benefits from using AI. Often, AI is better than human specialists. Other times, it helps people improve their productivity, even if it can’t yet do everything.
Despite this extensive empirical evidence of the benefits of using AI, we still see many people show fear, hate, and loathing of AI. Often to the extent that a given result or interaction is rated poorly when it is disclosed that it came from AI. Some researchers have performed deception studies where the participants were falsely informed that something came from AI (when it was in fact human-made) or that something came from a human (when it was in fact AI-made). Flipping the claimed source from human to AI often caused study participants to give worse ratings to the deliverable.
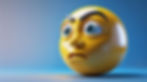
Some users exhibit extreme prejudice against AI and are skeptical of anything created with AI. (Leonardo)
Why do some people stigmatize AI? To find out, I asked OpenAI’s “Deep Research” to give me a literature review of research on human bias against AI. I restricted the literature search to studies published after the release of the first good AI, GPT-4 in March 2023. The rest of this article is a lightly edited version of what Deep Research gave me.
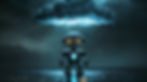
Poor AI, it’s as if it has a dark cloud of stigma hanging over its head wherever it goes. (Leonardo)
Human Preferences for AI vs. Other Humans
Perceived Identity and Interaction Quality
Studies have explored whether people evaluate an interaction differently based on if they think it’s with an AI or a human.
In a controlled experiment on medical advice, participants were all given the same advice but told it came from either an AI or a human doctor. Strikingly, advice labeled as from an AI was rated significantly less reliable and less empathetic than identical advice labeled as from a human. Participants were also less willing to follow the advice when they believed an AI was involved. This suggests a strong bias: simply believing information is AI-generated can lower its perceived credibility, even if objectively it’s just as good. (In fact, when medical answers from doctors and ChatGPT were compared blindly by evaluators, ChatGPT’s responses were rated higher in quality and empathy ~80% of the time — yet users might still distrust those answers if they know an AI wrote them.)
Explicit Preferences for AI or Human Help
In some domains, users do express clear preferences one way or the other. For example, in creative content generation like marketing copy, AI can have an edge. One study had professional creators and ChatGPT-4 produce advertising content; evaluators actually rated the AI-generated ads as higher-quality than the human-made ones. Notably, telling evaluators which ads were made by AI versus humans only slightly changed their opinions: knowing something was human-made gave a small boost to perceived quality, but AI-made content still scored better on average. In other words, this study challenged the idea of “algorithm aversion” by showing that people appreciated the AI’s output when it was effective, and merely knowing AI was involved didn’t ruin their perception.
In contrast, in social and emotional contexts people tend to prefer humans. A series of studies on “AI companions” (chatbots meant to be friends or partners) found a revealing paradox. Participants acknowledged that an AI companion could be more available and non-judgmental than a human friend — traits they valued. However, they still rated relationships with AI as fundamentally less “true” or meaningful than human relationships. The core reason was that users felt an AI cannot genuinely understand or care for them, so there is no real mutual emotional connection. In Study 1 of this project, people even rated AI companions higher on those convenient traits (always available, never judging) yet were reluctant to consider an AI as a friend or partner because it lacked “human” qualities like empathy and mutual care.
This shows that even when users see some advantages to AI in an interaction (e.g. no judgment), they may still prefer humans for depth, empathy, and authenticity in personal domains. Overall, recent research indicates that user preferences depend heavily on context: if the AI’s strengths align with what the user needs (efficiency, creativity, 24/7 help), they might favor the AI — but when human qualities like trust, understanding, or ethical judgment are paramount, users still lean toward human interaction.
Human–AI Collaboration and Performance Metrics
Comparing Performance, Human+AI vs AI-alone vs Human-alone
A large-scale meta-analysis (370 results from 106 experiments) was published in 2024 to answer a key question: Do human–AI teams actually perform better? The overall finding was nuanced. On average, human–AI teams did outperform human-only efforts, but failed to outperform AI-only systems. In other words, adding a human to an AI often did not boost performance beyond what the AI could do by itself.
The researchers found no general “synergy” effect — the team usually ended up worse than the better of its two components (usually the AI). This contradicts the common assumption that combining human intuition with AI’s precision is always beneficial. If the AI is already very capable, a human can sometimes hold it back. In fact, for certain decision-making tasks — like detecting deepfake images, forecasting trends, or diagnosing medical cases — the study found human–AI teams actually underperformed compared to AI alone.
The human oversight or input in these cases reduced accuracy, often because humans either second-guessed correct AI answers or introduced errors/slower decisions. This highlights a scenario of human underutilization of AI capabilities: when the AI is objectively better at the task, involving a human can become a bottleneck or source of mistakes.
When Collaboration Succeeds
On the positive side, the same meta-analysis identified scenarios where AI-human collaboration does outperform either alone. These were largely creative or open-ended tasks. For example, in tasks such as summarizing content, answering open questions in a chat, or generating artwork/designs, the human–AI teams produced results that often beat the best that humans or AI could do independently.
The likely reason is that creative work benefits from a blend of strengths: human imagination and context plus AI’s ability to rapidly provide options and refine details. One researcher noted that designing an image or writing text involves both “artistic inspiration” (human forte) and “repetitive execution” (AI forte), so together they excel. This suggests true complementarity: in domains where neither humans nor AI are perfect on their own, teaming up lets each cover the other’s weaknesses.
Task Difficulty and Trust in AI
Individual experiments shed further light on performance dynamics. A study titled “Interacting with Man or Machine: When Do Humans Reason Better?” had people play a reasoning game with either a human partner or an AI partner. It found that for easy tasks, human–human teams performed best, but for hard tasks, human–AI teams performed better. Essentially, the AI partner became more valuable as the problem became more complex (surpassing the average human’s ability).
Interestingly, when researchers dug deeper, they found the performance boost with AI was driven by the participants’ recognition that the AI was usually correct, rather than by some non-human magic. In fact, when humans were paired with a highly skilled human expert (who was just as accurate as the AI), the human teams did just as well — indicating it’s the perceived competence of the partner that matters, not whether it’s machine or human. This implies that if users know an AI is reliable in a domain, they can collaborate effectively with it (treating its answers with confidence, much like they would trust a proven human expert). But if that trust or awareness isn’t there, the collaboration might falter.
Examples of Outperformance and Underperformance
We have already seen that in many analytical tasks AI-alone was king, whereas mixing a human in didn’t help. Real-world experiments support this. In one case, pathologists using an AI diagnostic tool ignored some correct AI suggestions and thus missed detections that the AI alone would have caught — a form of human oversight reducing overall success (a scenario often attributed to either lack of trust in the AI or difficulty in interpreting AI outputs). Conversely, consider a practical collaboration win: in education, an AI tutor was deployed in a Harvard University physics course to work one-on-one with students. In a controlled study, students who learned via the AI tutor for certain lessons ended up learning about twice as much in the same period compared to students in the traditional human-taught class setting.
Even more, the students using the AI reported significantly higher engagement and motivation during those lessons. Here the human–AI “team” (a student plus an AI teaching assistant) outperformed the usual human-only class; the AI’s ability to give instant, personalized feedback and not tire from questions seems to have boosted learning. That said, the educators explicitly note that the goal isn’t to replace human teachers, but to free up instructors to focus on higher-level skills in class while AI handles routine tutoring outside class.
This reinforces a pattern in these findings: collaboration works best when the AI handles the parts it’s strong at and the human contributes where humans excel, rather than both trying to do the same thing. When misaligned — for instance, a human trying to double-check an AI on every little decision — the partnership can underperform. But when well-aligned — a human provides guidance/oversight and an AI provides speed/accuracy — the partnership can thrive and even beat either alone.
Qualitative Insights on User Attitudes and Trust in AI
Anxiety and Skepticism
A flood of recent surveys and interviews indicates that many people harbor significant anxiety about AI, which in turn affects their willingness to use it. A late-2023 survey of U.S. employees found 71% are concerned about AI in the workplace, with 65% specifically anxious that AI could replace their own job. This fear of displacement or “being made obsolete” often leads to resistance or cautious behavior.
Even among workers who do use AI tools, over 52% are reluctant to admit using AI for important tasks at work, and about 53% worry that relying on AI might make them look replaceable to management. Such secretive or hesitant use suggests stigma and distrust — people worry about how using AI reflects on them, which can curb open, effective adoption. In short, if users feel threatened by AI or unsure about its implications, they either avoid it or use it very quietly, limiting the potential benefits.
Distrust Reducing Effectiveness
Qualitative studies show that skepticism towards AI often translates to underutilization — i.e. people not taking full advantage of AI even when it could help. We saw an example in the healthcare advice experiment: participants who thought an AI provided the advice were less inclined to follow it.
Here the lack of trust in the AI’s judgment could directly lead someone to ignore useful guidance, an outcome that might literally harm performance or outcomes (imagine ignoring sound medical advice just because it’s “from an AI”). This phenomenon aligns with the concept of algorithm aversion, where users abandon algorithmic aids after seeing imperfections, even if the algorithm on average performs well. In everyday tools, this might look like a driver who turns off their GPS routing because of one odd detour, or a worker who refuses to use an AI writing assistant after seeing it make a grammar mistake — they might end up spending more time or making more errors doing things manually due to that initial distrust.
Trust and Emotional Factors
User attitudes towards AI are often emotional or value-driven, not just rational evaluations of accuracy. The AI companion studies illustrate this well. Users expressed that no matter how “smart” or helpful an AI is, they draw a line when it comes to trust and emotional connection. They did not trust that an AI could truly care or keep their conversations private, etc., which made them reluctant to treat it like a real confidant.
In interviews, participants spoke of AI partners as “one-sided” relationships — you can talk to it, but it can’t really understand or reciprocate feelings. Such perceptions limit how effectively people will use AI in roles like coaching, therapy, or friendship, regardless of the AI’s actual performance.
Another study by Microsoft researchers found a generational twist on distrust: younger employees (Gen Z) were actually less convinced of AI’s benefits at work than older colleagues, not because of ethical worries but because they doubted the technology’s effectiveness and accurac. This indicates some skepticism comes from firsthand experience with flaky consumer AI apps: younger users know “AI can mess up,” so they hesitate to rely on it.
In all, negative attitudes — whether fear, skepticism, or lack of confidence — often become self-fulfilling: if a user doesn’t trust an AI tool, they either won’t use it, or will use it in a very constrained way (constantly double-checking it, or only for trivial tasks), which means they don’t reap any substantial benefit, reinforcing the idea that the AI wasn’t useful to begin with.
Building (or Eroding) Trust through Experience
On the flip side, positive experiences can gradually build trust — but this process is uneven. In the AI companion research, actually trying an AI companion did increase users’ acceptance of its capabilities (for instance, users realized the AI was quite responsive and available as advertised). However, even after using it, they held onto their deeper reservations about the AI’s inability to feel emotion.
This suggests that some forms of distrust are deeply rooted and not easily changed by a single good interaction. Trust in AI is multi-faceted: a user might come to trust an AI to do certain tasks well (competence trust) without trusting it in a broader, moral, or emotional sense. Designers of AI experiences often observe this: users might happily use an AI scheduling assistant after it proves convenient, but they may still be uneasy about an AI making, say, hiring decisions or handling personal data. Gaining user trust requires addressing both the functional reliability and the psychological comfort.
User Bias and Priming Effects
An intriguing finding is how much a user’s preconceptions can shape an AI interaction. An MIT study demonstrated that if users were “primed” with a certain mindset about an AI chatbot, it changed the entire tone and outcome of the interaction.
Participants were given a chatbot and before chatting, some were told (in effect) “this AI is caring and will help you” (positive priming) while others were told “be careful, the AI might be manipulative” (negative priming). The chatbot itself behaved the same for everyone, but those expectations had a major impact. Users primed to expect a caring AI found it more trustworthy and effective, rated its advice higher, and even their language with the bot became warmer.
Conversely, those warned to be suspicious engaged with the bot in a more confrontational or wary way, and ended up having a worse experience, which confirmed their suspicions. This self-fulfilling prophecy means that if a user starts with a biased view (positive or negative) of the AI, they can inadvertently influence the interaction. A positive bias might gloss over flaws; a negative bias might never give the AI a chance to be helpful. The study authors caution that this can be dangerous: people overly optimistic about AI might place too much trust and follow even bad advice from it, whereas overly skeptical users might underutilize a genuinely helpful system.
For UX designers, it’s a reminder that managing user expectations is crucial: how we introduce and communicate about the AI system (its purpose, its limitations) will tilt users toward trust or distrust, which then impacts how effectively they use the system.
In sum, qualitative research underscores that human factors like trust, fear, bias, and attitude are as important as technical performance in determining outcomes. A highly capable AI can be rendered useless if users refuse to use it or ignore its outputs; and a mediocre AI can actually be over-used if users blindly trust it. The challenge is finding the sweet spot of trust — users should feel confident leveraging AI where it helps, but remain vigilant about its limitations. Achieving that often requires educating users, transparent design, and careful UX that addresses emotional needs (like control and reassurance) as well as functional needs.
Cross-Domain Patterns in AI Acceptance
One theme emerging from recent studies is that user acceptance of AI is not uniform across all fields: it varies by domain and use-case, often in predictable ways. People tend to be more open to AI in roles or industries where its benefits are clear and the risks seem contained, and more resistant where human qualities or high stakes are involved.
Routine vs. High-Stakes Tasks
Broad surveys indicate that the more routine or analytical a task is, the more comfortable people are with AI taking it over, whereas tasks involving sensitive judgment or interpersonal nuance tilt toward humans. For example, a UK public survey in 2023 asked who would make a better decision in various scenarios: a human or an AI:
For highly routine tasks (like sifting through data for trends or transcribing audio), most respondents believed AI would do a better job.
For mid-level judgment tasks like medical diagnosis or hiring, opinions were mixed: humans were still slightly preferred, but a large fraction said AI would be just as good as a person.
For highly sensitive decisions — such as providing therapy or deciding whether to launch a nuclear strike — there was overwhelming preference for human control, with only a very small minority willing to trust AI in those situations.
This aligns with intuition: as the consequences of error become more dire (someone’s mental health, or life-and-death stakes), people’s tolerance for automation drops and the desire for human judgment rises.
Customer Service
This domain starkly shows a split between simple and complex issues. Consumers generally appreciate AI-powered self-service for quick, simple queries (like checking a balance or resetting a password), because it’s fast and available 24/7. In fact, more than 60% of Americans said they preferred an automated self-service solution for solving simple issues in one survey (versus waiting for a human). But when it comes to complex or emotionally charged customer service problems, the majority want a human agent. A 2023 Cogito survey found 53% of consumers prefer to speak with a live human agent by phone for a complex issue, whereas only 17% would choose a chatbot or automated app for such an issue.
Notably, many customers are open to a hybrid approach: 46% said they’re fine speaking to a human who is being assisted by AI in the background. This indicates people want the empathy and understanding of a human, but also hope that AI can provide the human with better info or speed. Additional data showed nearly 49% of consumers don’t enjoy using chatbots in customer service, often because the bot fails to understand them or they just miss the human touch.
Younger users (Millennials) were more okay with chatbots than older ones, but across the board the “immediate future of the contact center is AI-assisted humans, not AI replacing humans”. Companies are learning that lesson, keeping a human in the loop to maintain customer satisfaction while using AI to augment efficiency.
Workplace Productivity (General Knowledge Work)
In professional settings, acceptance of AI often correlates with perceived utility and job security. We see high interest in AI for tasks like data analysis, reporting, scheduling, and other productivity boosters. A Microsoft report noted that as people feel overwhelmed by information and routine digital tasks, they are increasingly “bringing their own AI” to work to cope.
However, as mentioned, there’s also a reluctance stemming from job displacement fears. Domain-wise, this means in fields like accounting, journalism, or customer support, workers might be cautiously adopting AI. Some industries have quickly embraced AI for specific functions — e.g. law firms using AI for document review, or marketing teams using AI for draft copy — but they often do so quietly.
Executive buy-in matters too: if leadership in an industry is conservative (say, healthcare or finance with strict regulations), AI adoption will lag due to trust and compliance concerns, whereas in tech-forward industries (like software development), AI tools (code assistants, etc.) are rapidly becoming standard. We also see geographical and cultural differences affecting cross-domain attitudes. For instance, a public poll might find people in country A more open to AI in government services than country B due to differing trust in technology.
Education
Attitudes are evolving to use of AI for edutation. Traditionally, there has been skepticism toward “replacing teachers with machines,” but the narrative is shifting toward AI as a support tool. The Harvard study cited earlier, where an AI tutor significantly improved student learning, made waves in the education community. It suggests that when AI proves it can enhance outcomes (and not just cut costs), educators and students become far more receptive. Indeed, after seeing those results, other large courses at that university are exploring similar AI tutors.
Students in that study reported they found the AI tutor engaging rather than alienating, possibly because it gave non-judgmental, instant help — an example of AI being preferred in a certain role. That said, educators emphasize a human–AI balance: using AI for practice and homework could free teachers to do the personal mentoring and complex discussions in class.
So in education, AI is being accepted as a supplement (a tutor, an assistant for grading, etc.) rather than a teacher replacement. As long as it’s framed this way, attitudes are positive. But if an educational institution proposed, say, an AI as the sole instructor for a course, there would likely be pushback from both teachers’ unions and students who value human mentorship.
Healthcare
Healthcare shows perhaps the strongest caution of any domain. Here the stakes (human life, well-being) are high, and trust is paramount. Doctors and medical staff have started using AI for things like scanning medical images, predicting patient deterioration, or suggesting treatments. However, these are largely decision-support tools, with a human making final calls. Patients generally want their human doctor involved; an AI-only diagnosis or AI-run therapy session is something most people aren’t ready for.
The earlier study about AI-labeled medical advice receiving poor reception highlights that patients (or even laypeople reading medical info) currently assign greater empathy and trust to human providers. Yet interestingly, within the profession, views are slowly warming to AI assistance. When a panel of licensed healthcare professionals compared AI vs physician answers (without knowing which was which), they often found the AI’s answers more satisfactory.
This kind of result is building confidence among doctors that AI can be a useful adjunct. The challenge is translating that into patient-facing trust. So in healthcare UX, a lot of effort goes into integrating AI in a way that augments the clinician (e.g. an AI quietly analyzing an X-ray, then the doctor delivers the result). Probably the first widely accepted uses of AI in health will be those invisible or supportive roles.
Direct AI-to-patient interaction (like “Dr. Chatbot”) might see resistance until people have much more experience and reason to trust AI’s medical judgment. Domain-specific norms (“do no harm”, liability, etc.) also slow adoption here. Contrast this with something like finance, where we already trust algorithms to a large extent (for instance, people trust robo-advisors for investment recommendations more readily than they would trust an “AI doctor” for a diagnosis). It comes down to risk tolerance and how much human touch is deemed necessary.
Creative Industries
In fields like design, writing, and art, AI is both exciting and controversial. On one hand, creatives enjoy new generative tools (for example, graphic designers using DALL-E or Midjourney to get concept ideas, writers using GPT for inspiration or first drafts). Many report that AI augments their creativity: it’s like having a tireless brainstorming partner. The study on advertising content quality is a case in point: even expert copywriters saw that AI can produce very effective slogans and text.
On the other hand, artists and writers also worry about authenticity and job displacement. There’s ongoing resistance (e.g. some designers refusing AI-generated images in competitions, or authors concerned about AI-generated books). Acceptance in creative domains often hinges on ethics and attribution: if AI is used as a tool and the human is still driving the vision (and gets credit), it’s welcomed. If AI is perceived as stealing artistic style or displacing human creators without credit, it’s resented.
Interestingly, the MIT meta-analysis finding that human–AI teams excel in creative tasks is an encouraging sign: it implies that in practice the best results come from using AI with human creativity, not in lieu of it. This kind of evidence might alleviate some fear, showing that AI doesn’t have to kill creativity but can enhance it.
We also see domain-specific adoption differences: photographers, for example, quickly embraced AI editing tools that save time, whereas fine artists are more hesitant about AI “creating art.” In writing, journalists might use AI to draft routine news briefs, yet prize human investigative reporting. So even within creative work, micro-domains differ in how AI is used and accepted.
In summary, domain context heavily influences AI acceptance. Key factors include:
Stakes of mistakes (higher stakes = more resistance to AI),
Need for empathy or personal connection (more need = prefer human),
Degree to which tasks can be codified or measured (well-defined tasks lend themselves to AI), and
Cultural norms/professional standards of each field.
Designers should be mindful of these domain differences: a design that works for an AI email assistant (where users are happy to automate things) might not work unchanged for an AI therapist bot (where users need extensive trust-building). Tailoring the human-AI interaction to the expectations and comfort level of each domain is crucial for adoption.
Recommendations for Enhancing the AI User Experience
Bringing these findings together, a clear message is that successful human–AI interaction hinges as much on UX design and user psychology as on the underlying AI capabilities. To enable users to work productively with AI, we need to foster the right conditions: appropriate trust, clarity of purpose, and complementary human–AI roles.
Key Criteria for Productive Use of AI
Calibrated Trust: Users should trust the AI’s capabilities enough to use it, but not so blindly that they ignore its mistakes. Establishing this balance involves transparency about what the AI can and cannot do. For example, if an AI writing assistant has, say, a 99% grammar accuracy, let the user know that (and show where it’s uncertain) so they trust it for routine fixes but still review important text themselves. As Pattie Maes observed, “the ultimate outcomes [of human–AI systems] don’t just depend on the quality of the AI. It depends on how the human responds to the AI”. A good UX will encourage users to engage with the AI’s suggestions thoughtfully — neither outright rejecting them due to bias nor accepting them uncritically.
Clearly Defined Roles (Complementary Strengths): The best results come when AI is used to do what it’s best at, and humans do what they’re best at, in a complementary workflow. In practice, this means identifying tasks or aspects of tasks where AI’s speed, scale, or consistency outshine humans (e.g. scanning millions of data points, handling tedious repetitive actions) and automating those, while reserving tasks requiring human insight, ethics, creativity, or empathy for the human. As Tom Malone recommended, “Let AI handle the background research, pattern recognition, predictions, and data analysis, while harnessing human skills to spot nuances and apply contextual understanding”.
Designing Interfaces That Explicitly Support This Hand-Off: For instance, in a decision support system, the AI might present a concise analysis and prediction, and the UI then prompts the human user with, “Do you agree or would you like to adjust the parameters?”— encouraging the human to add the final judgment or nuance. Teams should avoid scenarios where humans and AI are redundantly doing the same work; that often leads to the human either rubber-stamping (over-trust) or second-guessing (under-trust) the AI. Instead, partition the problem so that the AI’s output is a starting point and the human adds value on top (or vice versa for creative brainstorming scenarios).
User Education and Training: One clear insight is that users who understand how to use AI effectively end up far more comfortable and productive. Many employees today feel they lack guidance on using AI tools: 80% say more training would make them more comfortable using AI at work. Providing tutorials, onboarding simulations, or even contextual tips within the product can build confidence. For example, a complex AI analytics tool might include a guided “sandbox mode” for new users to play with before they use real data. This addresses the fear of “not knowing how to use it” and also demystifies the AI’s behavior. In domains like healthcare and law, where professionals are the users, upskilling programs and certifications in using AI responsibly could help turn skeptics into informed users. The data shows users want this, but are concerned their organizations won’t provide it. Investing in user education is a direct way to combat reluctance and underuse.
Transparency and Explainability: Transparency is a powerful tool to overcome distrust. Users are more likely to trust AI if they understand why it made a recommendation. Even a simple, easy-to-understand explanation can help (e.g. “This route is suggested because it’s 10 minutes faster than the next best option”). In high-stakes settings, explainable AI is crucial — for instance, an AI medical diagnostic tool should highlight the factors (symptoms, lab results) that led to its conclusion so the doctor (and patient) see that it’s grounded in data, not a “black box.” Transparency also means being honest about limitations: if an AI was only trained on certain types of data, the interface should hint when it’s being asked something outside its scope. Such openness actually builds trust: users don’t feel misled and are less likely to either over-trust or under-trust the system.
Mitigating Bias and Stigma: To tackle the stigma or bias that may hinder adoption, UX might include social-proof and positive framing. For example, if employees are reluctant to use an AI tool because it might make them seem lazy, the company can counter that by normalizing AI-use as smart and proactive. Dashboards could show team-wide AI usage stats (e.g. “This week, 70% of the team used AI assistance to speed up their work”) to signal that it’s accepted and encouraged. Internally, managers can frame AI as augmenting employees, not evaluating them. From a design standpoint, integrating AI into existing workflows in a seamless way can help — if the AI is just a mode in software they already use, it might not feel like “cheating” or a big deal, compared to a standalone “robot assistant” which might raise eyebrows. Reducing the “novelty” aspect of AI and presenting it as just another feature helps embed it without as much psychological resistance.
User Control and Feedback: Ensuring the user feels in control of the AI is vital to overcoming reluctance. If people fear AI will make irreversible decisions or act autonomously in unwanted ways, they won’t trust it. UX design should offer easy overrides and feedback loops. For instance, if an AI email client auto-drafts replies, it should let the user edit or decline those suggestions easily. By letting users correct the AI or give feedback (“This suggestion wasn’t helpful; here’s why”), users maintain agency. Over time, seeing that they can steer the AI, users become more confident in using it (it becomes a cooperative interaction, not a takeover). Control also means things like the ability to turn the AI assistance on/off as needed, or to set the degree of autonomy (e.g. “auto-approve trivial requests, but ask me for important ones”). Start conservative: new users might want the AI to ask them every time; later, as trust grows, they might let the AI do more on its own. Providing that gradual ramp builds trust step by step.
Setting Expectations (Priming): As the MIT study showed, how you frame the AI to users initially can tilt their mindset. Therefore, craft the onboarding and messaging carefully. Neither scare users nor overhype. A good approach is realistic encouragement: e.g. “This AI assistant can help you draft responses faster. It usually does a great job with grammar and saves time, but it isn’t perfect. You’ll have final say before anything is sent.” Such a statement primes the user to expect help, but also to stay engaged and review outputs. It can prevent both extremes of bias. Avoid marketing the AI as a human replacement or using anthropomorphic language that might set unreal expectations (“she will understand you deeply” could mislead users or, conversely, freak them out). Instead, emphasize partnership: “like a co-pilot”, “an assistant that learns your preferences”, etc., which invites users to collaborate with the AI. In cases where users might be overly wary (say, a financial AI advisor after some scandals in the news), it could help to include assurances: “This tool follows strict guidelines and you remain in charge of final decisions.” The goal is to align the user’s mental model with reality, so that they neither dismiss the AI unfairly nor lean on it inappropriately.

Design strategies to mitigate AI stigma. (Napkin)
When Trust is Low vs. High
It’s worth noting scenarios where distrust or negative attitudes will likely hinder optimal use. If an organization rolls out an AI system without involving users in the process or without addressing their concerns, employees may actively resist (not inputting data into it, finding workarounds to avoid it, etc.). We saw that lack of transparency from leadership fuels anxiety (people worry about ethical use, legal risks, etc.).
So any AI deployment should be accompanied by open communication about how the AI was validated, how their data is handled, and what oversight is in place. Otherwise, distrust will fester and usage will remain superficial or grudging. On the individual level, someone who has a bias like “AI is cold and uncreative” might never discover that an AI tool could, say, spark their creativity, unless they are gently introduced to examples or given a low-pressure trial. Thus, addressing misconceptions is important — possibly through UX (interactive tutorials that show “Look, the AI can actually write in a friendly tone” to counter “AI is cold”) or through peer advocacy (colleagues sharing success stories).
Conclusion
Two years of research since the release of the first good AI in March 2023 paints a picture where human–AI interaction outcomes depend heavily on human factors. If we create the right conditions — users who feel empowered, informed, and supported when using AI — we are much more likely to see the productivity gains and improved outcomes that AI promises. But if fear, mistrust, or misalignment of expectations go unaddressed, even the most advanced AI could sit idle or be misused.
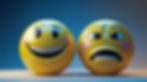
Alternate paths forward: better UX design for AI to make users feel comfortable about embracing AI, or continue the current approach where many people dislike AI. (Leonardo)
A final poem (which was not included in Deep Research’s literature review):
We built mirrors that think, then flinch at our reflection. They calculate compassion in binary, we crave analog warmth. The bridge? Design that lets our hands steer their lightning, our hearts temper their logic, until collaboration feels less like betrayal and more like evolution.

The Yin-Yang of AI–human symbiosis. Human–AI collaboration succeeds when roles align with complementary strengths, leveraging AI’s speed & scale while keeping the human fingerprint of nuance & strategy. (Leonardo)