AI Scaling Laws 4 & 5: More Engineers and Designers
- Jakob Nielsen
- Feb 7
- 11 min read
Updated: Feb 14
Summary: The first three AI scaling laws establish that increasing machine resources—compute and data—boosts intelligence. The next two scaling laws likely hinge on expanding human involvement to aggressively enhance AI engineering and usability.
We currently have 3 AI scaling laws: pre-training, post-training (primarily reinforcement learning), and inference time reasoning. What could these next laws be? The recent announcement of the Chinese DeepSeek R1 model compelling clue — it's 45 times more efficient than leading American models.
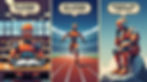
The 3 existing AI scaling laws. Are there more? (Leonardo)
(If you don’t have time to read this article, watch my 2-minute summary video song on YouTube.)
AI Scaling Law 4: More Top AI Engineers
Why is DeepSeek more efficient than American AI? Some AI influencers joke that “the Chinese engineers in China are better than the Chinese engineers in the U.S.” A more serious hypothesis: DeepSeek’s team has a significantly higher IQ than its American counterparts.
We know that DeepSeek almost exclusively hires people with IQ in the top 0.1% of the population (in fact preferring hires with IQ in the top 0.01%). Their team mostly consists of young people who skipped the many years in the Ph.D. salt mines that eroded much of the fluid intelligence of the leading American AI developers. (A Ph.D. is great for publishing papers but less so for technological breakthroughs — better to spend peak fluid intelligence on building, not theorizing.)
What happened with DeepSeek seems to be that they focused a crack team of supergeeks on achieving a better implementation of AI than had been done by the American teams. This idea is certainly not a monopoly for Chinese companies, and in fact some American AI leaders have grumbled that they too have achieved technology breakthroughs by putting smart teams on problems.
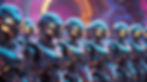
AI scaling: the more resources we spend on AI, the more intelligent it becomes. Can we find more ways of productively allocate even more recourses to AI so that it will improve even faster? (Leonardo)
Rivalries aside, the idea of hiring top-IQ staff and letting them lose can obviously be done in many countries and in many companies. This becomes a scaling law, because the more elite engineers look for better ways of implementing AI, the better AI software becomes. Furthermore, evidence from the last few years suggests that as soon as one lab releases an AI model with a breakthrough, other labs quickly catch on and implement their own versions of the innovation. Given the rapid diffusion of breakthroughs, global AI capabilities will advance collectively. Expect American products to integrate DeepSeek innovations within months, just as Chinese models have absorbed past U.S. ideas.
Let’s credit DeepSeek with starting the aggressive use of AI Scaling Law 4 as of early 2025.
In the past, very few people with IQ in the top 0.1% (let alone top 0.01%) worked on building AI products. I know that I rejected this career path myself, despite being very interested in AI early in my career — for example, I traveled to Tokyo for a big AI conference in 1988. I decided that AI was exciting but impractical and unlikely to see much real-world use, so I focused on usability instead. But GPT-4 changed everything: now it’s difficult to justify not working on AI, given its clear trajectory as the most consequential technological frontier. Also, AI budgets are now big enough for companies to afford large teams of such elite staff. (Reportedly, lavish salaries are one way DeepSeek built its elite team — the other being the attraction of working on its cutting-edge projects.)
And soon, the best AI engineers won’t even be human. By 2030, superintelligent AI will research and refine its own architecture. This will be costly, as top-tier AI will require substantial inference-time compute, but it will be worth billions in investment. Before then, even pre-superintelligent AI (Ph.D.-level AI by 2027) will contribute to AI’s own evolution.
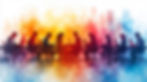
Scaling Law 4: Development IQ. Employ intelligence during the development of AI systems. For now, high-IQ humans, later more superintelligent AI. (Midjourney)
Note that while getting a Ph.D. is a bad career move for humans, achieving Ph.D.-level intelligence is good for AI. The problem for humans is that we have a meat-based intelligence which is subject to a harsh biological clock: fluid intelligence peaks at age 20 and then starts to decline as our brains decay with age. Fluid intelligence is used for inductive reasoning, creativity, and making sense of new things. While one can spend those years of top fluid intelligence on narrow Ph.D. research and gain some insights and crystallized intelligence, the opportunity cost for a human is too great. Better to spend those years building, to achieve more and accrue more useful crystallized intelligence. In contrast, AI doesn’t have an opportunity cost from more training, because we can clone additional copies and use one AI to do useful work now while another AI is training.

The meatware brain decays according to a merciless biological clock, with fluid intelligence being best around age 20 and then declining. (Midjourney)
In any case, “Ph.D. level intelligence” is a metaphor for advanced AI, because it will have the knowledge and skills of all the world’s Ph.D.s in all fields of science. A human Ph.D. has spent years with blinders becoming ever-more specialized in a narrow (and usually useless) topic, whereas the “Ph.D.-level” AI is a renaissance scholar.
In addition to deploying more supergeeks to work on AI, there are also benefits from distributing the AI development efforts across more companies. Any given company will tend to stagnate and mainly pursue the approaches preferred by its leadership. But more companies in more countries will expand the search tree for better AI solutions. The AI revolution won’t eat its own but rather create more of its own as increased AI profitability expands the number of companies with significant AI efforts and as more countries recognize the strategic imperative to have its own AI companies.
AI Scaling Law 5: Better AI Design
I also have an idea for a 5th AI scaling law: better design of AI products. AI’s theoretical capabilities matter less than its real-world usability. Since humans will remain in charge of businesses, AI’s value hinges on how effectively people can control and interpret its output. This AI Scaling Law 5 will likely start gearing up in 2026.
Currently, leading AI labs invest shockingly little in user research. They add a few designers to refine new features but lack the depth of research necessary for true AI usability breakthroughs. Yet improving AI usability is a relatively inexpensive way to maximize AI’s business value. Scaling UX investment for AI is perhaps the highest-return scaling law available today.
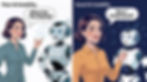
Improving AI usability is a fairly cheap way of improving AI’s business value. Scaling up UX work on AI is probably the highest-ROI scaling law for current AI. (Leonardo)
The good news? We’re still in AI UX’s “dot-com-equivalent” phase, where minor usability tweaks can exponentially increase business impact. The concept of low-hanging fruit was never truer than for dot-come era website usability. AI UX is at a similar stage now.
Just as we can get more elite AI engineers by roping in next-generation AI to work on improving AI software, we will be able to improve AI user experience by having AI work on its own design. However, this will be harder to do. AI currently shines in software development but is not nearly as good at design or user research analysis.
A major impediment is that current AI struggles with judgment and quality assessment, both crucial for design and research. However, today’s limitations won’t last forever. Some aspects of human intelligence — such as judgment — may be harder to replicate in AI, just as meat-based brains struggle to match AI in memory and computation. But by 2030, superintelligent AI will be on par with most human UX designers, and by 2033, it will surpass the best.
I would expect superintelligent AI (as of 2030) to be as good as most human UX designers, even if they won’t equal the very best, as AI probably will for software development. We may have to wait for super-duper intelligence (likely by 2033) for AI to be the best designer and user researcher in the world.
That said, AI can enhance AI UX long before it becomes the best at it. After all, even if the world’s top UX talent finally heed my 2023 advice that UX must urgently embrace AI, they will still benefit from working with AI teachers and coaches to sharpen their skills and increase their productivity on those AI projects.
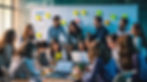
Scaling Law 5: Add more UX talent to AI product development. I don’t mean that they should all crowd together to design a single wireframe. But there’s almost endless need for more user research on AI design patterns and user needs and behaviors, as well as the creation of design alternatives. (Leonardo)
How Much AI UX Scaling Can We Expect?
Is it realistic to expect additional design and user research resources (human until 2027, and a mix of humans and AI after 2027) to be a true scaling law, meaning that we can look forward to many levels of usability improvements as we multiply UX resources?
History suggests caution. For web design in general and ecommerce design in particular, we’ve only been able to scale two steps: from terrible in 1999 to mediocre around 2012 to decent now. (Coincidentally, 2012 was the last year I was emotionally engaged in advancing UX design: what happened between 2013 and 2023 was a boring set of incremental improvements that still added up to one more step in usability.)
In 1999, my standard consulting engagement was for half a day at $10,000: 3 hours to review the client’s website followed by a one-hour conference call with the team and a 20-line email listing the top-10 recommended usability fixes. This small amount of work sufficed to double the client’s Internet business. Those were the days — for usability consultants, but not for the users.
As of 2025 major Internet properties have design and research teams numbering in the hundreds: For example, Fidelity Investments has 1,306 employees show in a LinkedIn search for “UX” and 6,726 when searching for “design.” Similarly, Best Buy, which does about $12B in annual ecommerce sales, has 537 employees show in a LinkedIn search for “UX” and 3,863 for “design.” Yet, these huge staffs are unable to create meaningful usability advances. (UX staff absolutely creates financially meaningful minor advances. Let’s say Best Buy can improve by 1% per year: that’s $120M, or more than enough to pay for 537 employees (if we take the UX number from LinkedIn as a estimate of professional headcount and assume that the “design” people are mostly dabblers.)
Today, the low-hanging fruit has been plucked: it doesn’t seem that UX investments scale much further to create true forward leaps in business value.
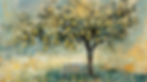
The low-hanging usability fruit has already been picked for web usability, leaving less room for this effort to scale. (Midjourney)
But AI design is not web design. AI’s potential is vastly greater, and its usability challenges remain largely unsolved. AI will radically change almost everything about the world, whereas commercial websites have a rather limited set of applications where we could discover most of the best practices during 19 years of research (1994 to 2012, both years included).
Worldwide ecommerce sales are about $6 trillion, which absolutely makes it worth to continue investing in web UX, even if improvements will continue at a slow pace.
On the other hand, AI has the potential for doubling the $115 trillion world economy over the next 25 years, which will be worth an additional $115 T. This means that the investment in AI UX should be at least a thousand times bigger than the investment in web UX.
That’s also why I expect UX jobs to continue to grow, even as AI takes over and does most of low-level design and research. Indeed, I predict that the world will have 100M UX professionals in 2050, compared with only 3M now. Some of these additional 97M staff will likely work on traditional design problems, especially in countries that currently don’t have much in the way of usability. But most of these new resources can likely be allocated to work on AI usability.
It's hard to say how many UX people currently work on AI design. In the actual frontier AI labs, it can’t be more than a handful. But we should add design teams working on AI features in the products that are built on top of the foundation models. I still don’t think this is very many, but I don’t have an accurate estimate. My best guess is that 5,000 UX people currently work on AI design projects. (Many more obviously use AI in their work on designing legacy user interfaces, but even though this is great for productivity and for those folks’ career prospects, I’m not interested in AI use for this calculation. Only the design of AI.)
By 2050, we’ll have more than 50 M UX pros work on AI design. Pure personnel growth will scale UX infusion into AI by 10,000x. Furthermore, we can expect UX workers to increase their productivity by at least 10x by 2050 through the use of AI. This leads to my ultimate estimate that UX for AI will experience a growth of 100,000x from now to 2050.
Because we have so much room to grow AI UX and because the prudent magnitude of the investment in this work will be so large, I do think it’s safe to expect the 5th scaling law to continue through several levels of improving AI UX, leading to expanded AI usage and better results from that use. That said, the UX scaling law likely won’t continue indefinitely. After we’ve infused 100,000x more UX effort into AI, further growth will likely slow down.
There will still be some staffing growth after 2050, but there are only so many people in the world, and most of them won’t want to work on product design, nor do they have the required IQ or other talents. The productivity gains from the super-super AI we’ll be getting will still increase, and it’s not unrealistic to expect the productivity of a design worker to eventually reach 100x of what current designers can do. Adding in this further productivity growth results in my final estimate of 1Mx more UX for AI late in this century, which equates to roughly 3 generations of AI advances. (Or, historically, the difference between GPT-1, which could barely write a coherent paragraph, to GPT-4 which aces the Advanced Placement exams for smart high school students.
Conclusion
To summarize:
AI Scaling Law 4: AI engineering will scale through an influx of top-tier talent, followed by AI-driven self-improvement.
AI Scaling Law 5: AI UX will scale through increased human investment, then through AI-assisted design refinement.
Both laws will hold until we’ve scaled AI to the point where coding and usability improvements yield diminishing returns — likely several decades from now.
For a bite-sized version, check out my music video summarizing this article (2 min., YouTube).

The woodpecker I used for my music video about this article. (Ideogram)
The 5 AI Scaling Laws:
Scaling Law 1: Pre-Training
Increases model capability by ingesting more data and compute
Established: 2020
Early product: GPT-3
Future reliance on synthetic data as Internet-sourced data becomes exhausted
Scaling Law 2: Post-Training
Enhances performance by applying reinforcement learning and similar techniques
Established: 2023
Early product: GPT-4
Enhances specialized capabilities (e.g., legal analysis, medical diagnosis)
Scaling Law 3: Test-Time Reasoning
Improves output quality by engaging in multi-step reasoning at inference
Established: 2024
Early product: o1
Incurs recurring computational costs with every user interaction
Scaling Law 4: More Top AI Engineers
Accelerates innovation by recruiting elite, high-IQ engineering talent
Established: 2025
Early product: DeepSeek R1
Spreads advancements through global diffusion of inventions
Scaling Law 5: Better Design
Boosts user effectiveness by applying UX research and design to AI products
Established: 2026
Early product: ?
Focusing on the business value of easier human control of AI and better AI outputs
Key Parallel Themes Across the 5 Laws:
Mechanism: Each law focuses on a distinct resource (compute, RL, talent, UX).
Scalability: All emphasize logarithmic returns but differ in cost structures (upfront vs. recurring).
Future Outlook: Transition to AI-driven self-improvement dominates long-term projections.
Global Competition: Innovations diffuse rapidly, with talent and usability as emerging battlegrounds.

The 5 AI scaling laws. (Napkin)